Although AI-driven marketing technology (martech) can be powerful, improper training, weak algorithm designs and hidden prejudices will quickly derail any campaign.
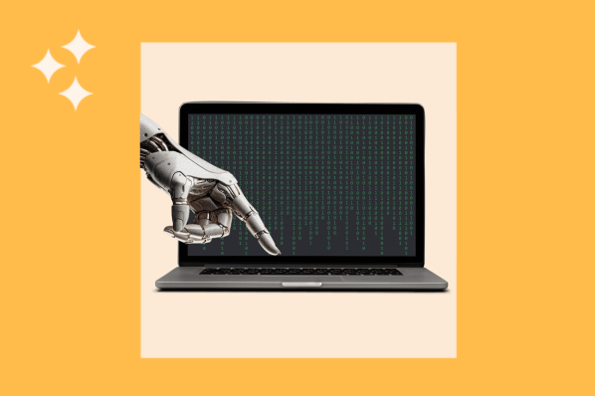
How can you identify and mitigate bias before it impacts your brand?
The Issue of Algorithmic Bias in Martech
Dove, the personal care company owned by Unilever, just became the first brand in its industry to pledge not to use generative AI in advertising. An accompanying two-minute video depicts search terms like “most beautiful woman” and “perfect skin” returning AI-generated models.
The video cuts from the uncannily flawless faces to real women, highlighting the impact of impossible beauty standards. This move is part of the Dove Campaign for Real Beauty, a 20-year campaign focused on showcasing all body, skin and hair types.
Dove may be the first to commit to such a pledge, but it likely isn’t the last. Its decision comes after various other brands have come under fire for experimenting with AI-generated content. Critics and consumers alike claim these tools favor certain stereotypes and demographics.
The Washington Post’s study on bias in generative content seemingly proves Dove’s point. The leading generative models — including Midjourney, Stable Diffusion and DALL-E — favored thin, light-skinned women when asked to generate a “beautiful” woman.
Nearly 90% of Midjourney’s images depicted light-skinned women. DALL-E and Stable Diffusion were barely better, with only 38% and 18% featuring dark-skinned individuals, respectively. This evidence suggests the potential algorithmic bias in martech is significant.
How Algorithmic Bias Impacts Marketers
Algorithmic bias in martech can influence consumers’ perception of a company. If it is glaring or consistent enough, it may impact a brand’s revenue, reputation or conversion rate:
- Poor Communication: Since 88% of website visitors are less likely to return after a bad experience, a chatbot that makes assumptions or displays prejudice is bad for business.
- Pointless Promotions: Customers may be shown irrelevant advertisements based on stereotypes instead of genuine browsing or buying behaviors, affecting sales.
- Inaccurate Pricing: AI-driven martech might use discriminatory pricing practices, charging certain groups differently based on stereotypes and favoritism.
- Irrelevant Recommendations: While a relevant suggestion makes customers 35% more likely to buy, irrelevant recommendations can impact revenue.
- Stereotypical Segmentation: A prejudiced model may skew characteristics’ weight, grouping customers into inaccurate segments based on clichés.
- Unreliable Predictions: Uninformed datasets result in ill-informed decision-making and financial losses. Data bias can result in up to 62% lost revenue, according to one survey.
Marketers who disregard the potential implications of algorithmic bias in martech may lose website traffic, anger customers and miss out on potential sales.
Methods for Identifying and Mitigating Bias
Generally, generative AI’s algorithmic bias is visible if you search for it. However, all kinds of models can produce prejudiced output — and it is often challenging to recognize. You should know how to identify it so you can mitigate it.
Your go-to method should be to audit your model’s data feed before integrating it into your martech. Minimizing the amount of stereotypes, assumptions and unfounded beliefs that get into training prevents it from learning to be unfair or bigoted.
A human-in-the-loop system is one of the most effective methods because it pairs one of your team’s marketing professionals with an AI. This person reviews its output for prejudice, ensuring only high-quality, relatively bias-free material makes it to consumers.
Another method involves analyzing your industry's historical and current state of bias. Since some use cases have a higher prejudice potential to specific communities than others, assessing the broader scope of prejudice can help you determine its likelihood.
No matter what type of AI you integrate into martech, you should ensure you develop a feedback loop. Since machine learning models learn based on feedback and interactions, inadequately addressing biased output reinforces it over time, perpetuating the problem.
3 Considerations for Fairness and Inclusivity
Bias will remain ever-present in marketing because it is a byproduct of societal norms and modern expectations. Prioritize fairness and inclusivity in your practices to minimize it:
- Diversify Datasets: Training AI-driven martech on a wide variety of text, images, audio and videos can help it learn to be inclusive.
- Be Transparent: Prioritizing transparency shows your customers you value fairness and strive to be an inclusive, ethical brand.
- Don’t Over-Personalize: Overpersonalization can cause a lack of content diversity, as well as user fatigue. In some cases, prioritize the user experience over hyper-personalization.
In today’s age, prioritizing fairness and inclusivity will get your brand far. Listen to customers’ concerns about AI and prejudiced martech to inform decision-making.
The Bottom Line of Bias in AI-Driven Martech
While nothing can be truly bias-free, you can come close if you regularly audit your model’s output, review your martech for accuracy and listen to feedback from your customers.
In an age of high AI concerns, diligence and consistency will result in better business outcomes.