Everyone wants the crystal ball or magic bullet that solves their problems or lets them know what the next best step is. Luckily for those of us who aren’t sorcerers, we have the statistical science of predictive analytics for business. Think of it as the natural human superpower of pattern recognition, but applied to huge data sources by AI and learning machines.
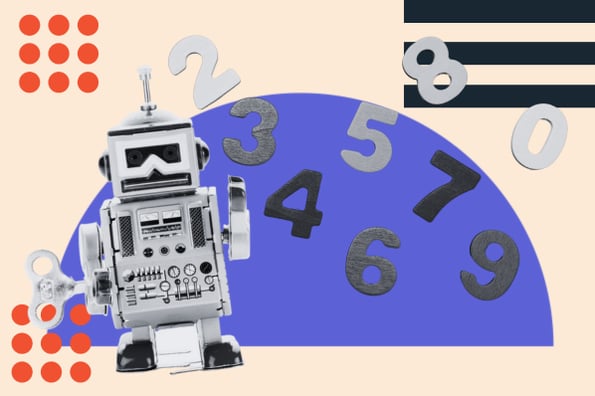
As a tool, predictive analysis only grows sharper as humanity collects more data. Having larger data sets over longer periods helps hone in on the predictions that help professionals make better, more data-driven decisions.
So, what can predictive analytics do for your business? It’s a relevant question across any number of industries, so let’s dig into the answers in order to do better business now and in the future.
Skip to:
- Industry Use Cases
- Common Predictive Analytics Models
- Benefits of Using Predictive Analytics
- Predictive Analytics Examples
What is predictive analytics?
Predictive analytics is a category of statistical science where current and historical data are examined in search of patterns that can help make predictions about the future.
Industry Use Cases
Any industry can use predictive analytics, yet for vastly different reasons and toward any number of desired outcomes. We’ve chosen three use cases that demonstrate very different ways predictive analysis has proven useful.
1. Healthcare
Use case: Predicting Risks, Benefits, and Outcomes
The healthcare industry uses data analytics in numerous ways to examine a patient’s disease process in context with historical data about the given disease. By detecting the patterns in the data, it’s both possible and useful to make a number of medically relevant predictions.
Let’s work through some uses for predictive analytics in regard to bariatric surgery. The US’ first nation-wide statistics on obesity became available in 1985.
Researchers used predictive analyses of their time on that data, which led them to predict that the risks associated with living with obesity would at some point become greater than the risks of surgery to treat the disease.
Later descriptive analysis (based entirely on historical data) found that the tipping point where that becomes true is when patients have a Body Mass Index of 40 or greater, or 35 or greater in the presence of a comorbidity. This is why Medicare will usually cover the cost of bariatric treatment for these patients.
Predictive analytics also had a role in predicting which health conditions could be indicative of an obesity disease process. It was later confirmed that common comorbidities of chronic obesity were found to include type 2 diabetes, obstructive sleep apnea, and a whole host of cardiac conditions.
Bariatric surgery data has been collected for decades now. Researchers reviewed that data in conjunction with new data about the effectiveness of gastric bypass surgery. They were able to make outcome predictions that gastric bypass surgery would not only reduce BMI but also treat other conditions.
They eventually discovered through two major studies in Utah and Sweden that patients who underwent this procedure achieved a 10-year reduction in cardiovascular morbidity of approximately 50%.
In other words, the control group that did not have the gastric bypass procedure was twice as likely to die of heart disease than those who treated their obesity surgically.
In comparison to the mortality rate of the gastric bypass procedure — a scant 0.24% — the statistics become part of a doctor’s own process for determining which recommendations they make to various patients. They predict when the benefits outweigh the risks in each case.
2. Entertainment
Use case: Content Recommendations and the Netflix Percentage Match
Every title you find on Netflix, Hulu, or Disney+ has data attached to it specifically for use in predictive analytics. The predictions they make with this data clearly have nothing to do with medical risks and benefits, serving a completely different purpose.
The entertainment industry uses predictive analysis to make their best guesses at what kind of entertainment will resonate with their various audiences and then which of those productions will appeal specifically to you. They look for patterns in what you watch and how you rank various titles, then run your data through their predictive algorithms.
The Netflix Percentage Match offers viewers a glimpse of the company’s predictions. As you browse their offerings, you’ll find a percentage just under the show title on the left.
They’ve used predictive analysis to show you how much they think you will like that show, based on how closely it resembles other shows or movies you’ve watched and/or given a thumbs up to.
3. Marketing
Use case: Effective Customer Segmentation
Marketers use predictive analytics for many reasons, including campaign planning by customer segment. In this use case, the data is examined for patterns to help predict which customers a campaign will be most effective on. Marketers can then target their audiences accordingly for best results.
Let’s take U.S. geographic areas, for example, and the preference for Coke versus Pepsi. Data shows that the northern US prefers Pepsi while the southern US prefers Coke — even though both products were created in the south. This regional divide is due to Pepsi’s acquisition by a northern Wall Street pioneer who owned Pepsi for a time and advertised aggressively in the north in the 1940s.
Modern marketing teams for Pepsi looked more closely at the old and new data. They then predicted that an effective Pepsi campaign could be targeted in the Appalachian region of the southern U.S. where Pepsi was originally created. They used the patterns they found in ever-growing data to their advantage and began their “Born in the Carolinas” campaign.
Something important that marketers should be mindful of are the programs, algorithms, and data sets they are using in their predictive analyses. Depending on what you use to examine the data or how large/inclusive your data sets are, you might discover all sorts of patterns — some useful, some not.
For example, AI programs used for performing predictive analysis will be better trained to recognize certain types of patterns than others. It’s up to marketers to decide which patterns they will use to sort their customers into effective segments. They may need to swap out or train up their AI analytics tools to extract more relevant and/or accurate predictions from their data.
Other Predictive Analytics Examples
Here are more examples of ways that various industries are using predictive analysis to work for them:
- Forecasting holiday inventory based on sales patterns from past holidays and customers’ current buying behaviors.
- Determining marketing trends in social media to plan future content.
- Improving virtual assistants like Alexa and Siri to better meet the needs of each user based on how, why, and for what they use their assistance.
- Weather forecasting — i.e., identifying patterns in how climate change is affecting storm movement and severity.
- Accurate credit scoring based on each consumer’s buying patterns and debt payments.
5 of the Most Common Predictive Analytics Models
There are a variety of predictive analytics models and algorithms being developed to process data. This list will surely grow in the years to come as people get more familiar and proficient with AI and machine learning. Let's review five of the most common models today.
1. Classification Model
Simple, flexible, and to the point, classification models are best for answering yes or no questions or describing relationships between the data in your dataset. Datasets are easily swapped in and out, so it’s a great place to start understanding the basic processes of predictive analytics.
Classification models are useful for answering questions like, “Are there patterns in this data that signify similar groups?”
It can also identify if-then situations like a decision tree: “Does this data indicate that if Y is true, then X — or if Y is false, then Z?” It requires you to ask for a certain output based on the input, and is thus categorized as supervised machine learning.
2. Clustering Model
Clustering is a great way to make discoveries within data. Instead of asking, “Are there patterns in this data that signify similar groups?” you would simply be provided with the data groups that have similarities. The groups may surprise you and be a breakthrough, or they may be related in ways that are not useful to you.
You would then decide what to do with the groups. For example, a marketer may feed the model customer data, and it may find that there are similarities between various customers that make them ideal candidates for social media ads as opposed to a separate group of customers that are more likely to see television ads.
The clustering model doesn’t require direction to produce outputs based on data, making it a type of unsupervised machine learning.
3. Time Series Model
This predictive model uses time as its metric. It considers historical and current data about variable details as they shift to predict those variables in the future. It’s best used for identifying and optimizing trends, cycles, and seasonal differences.
For example, a new florist opens a shop and stocks the sales floor with vases and fresh flowers but doesn’t know how much extra stock to have ready in the cooler on any given week. She doesn’t want to over-order because then the flowers may go bad before she can use them. She doesn’t want to under-order and run out of stock if there’s a pop in business, either.
If she opted for data-driven ordering, she could use a time series model with data from a similarly sized and frequented flower shop to order more accurately each season of her first year in business.
In January of the next year, she would feed the model her own data from her first year in business. This way, she can more and more accurately do her ordering each season.
4. Outlier Model
This model is a good one for solving problems by tracking an anomaly. Alternatively, it can help you identify a pattern among anomalies in the data set that may signify a predictable result.
An internet provider is a good example of a business that could benefit from this model. Your data shows a pattern of internet outage alerts last year, leading to a widespread need for home visits and line maintenance. The next time you see that alert pattern, you know to prepare your team for a wave of support tickets.
If you discover that the service area had flooding both times you sent your crews out, you can reasonably anticipate hard rains will trigger the alert pattern. Now, you could make a smart choice with your budget to fortify that service area against water damage or invest in waterproof lines and connectors to use on new hookups in that area.
5. Forecast Model
This is the model for you when you need numerical predictions based on historical data that is also numerical. It allows you to include additional relevant inputs to have as accurate a prediction as possible with as little risk as possible from variations you didn’t account for in the model.
This high-use model is particularly useful for banks and businesses that constantly work with money, track investment portfolios, create budgets, provide financial projections for stakeholders, etc. If you need a numerical prediction based on numerical data of any type for any venture, you’re likely to use forecast models.
Benefits of Using Predictive Analytics
Predictive analytics can help your team make more informed decisions. Your results are data-driven and come with greater confidence/buy-in potential. You can forecast and dedicate resources with less risk. You have historical data that increases the likelihood of your predictions.
You can also use the information to improve your offerings. You may find fresh revenue sources when a new buying pattern is detected in the data. You can optimize products and services based on customer feedback data. You may also stumble across new customer segments from unexpected data patterns.
In the end, the benefits of using predictive analytics are really only limited by what creative uses you can find for the patterns your tools discover inside your data.
Drawbacks of Using Predictive Models
The primary concern with predictive models is that what they produce are predictions, not facts. It is critical to communicate to leaders that the information they are basing their choices on is a form of data-driven prediction. Sometimes, the statistical significance of your numbers will be larger and more reliable, but it’s never 100% factual.
Additionally, consumer behavior changes. Your models are only as good as the freshest data you can get. Sometimes, that data is hard to come by or expensive to obtain.
Always keep ethics in mind. The AI and machine learning programs that inform your prediction models/algorithms may have come to you biased, may require additional training, or may require frequent updates to remain as accurate as possible.
Getting Started
There are an infinite number of ways that predictive analytics can be useful to your business. Using AI to dig deeper for patterns in data makes the usefulness of predictive analytics as limitless as our imaginations. Will you and your team dream up the next creative application that boosts your success by making data-driven decisions?
Editor's note: This article was originally published in June 2014 and has been updated for comprehensiveness.