In a 2021 report by Experian, 95% of business leaders reported a negative impact to the business due to poor data quality. These effects range from negative customer experiences to a loss of customer trust.
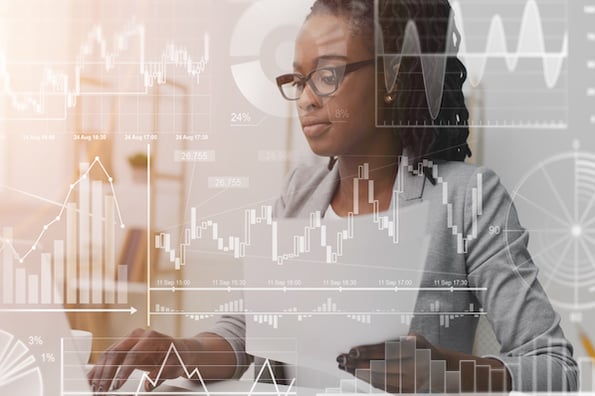
Both of these situations are bad news. But, the good news is that improving the quality of your data can reduce these negative impacts.
While it might seem like collecting data is half the battle, the real challenge is maintaining high standards of data quality throughout its entire lifecycle. In this guide, we show you best practices and examples of maintaining data quality so you can use it to make informed decisions about your business. Jump ahead to the sections that interest you most.
Characteristics of Data Quality
Data Quality Analysis & Metrics
What is data quality?
Data quality measures how well data serves its intended purpose as well as its accuracy and relevancy. The goal of having high-quality data is to make empowered, informed, and data-driven decisions to improve your business.
How does quality data empower good business decisions?
Let's take a step back and review an example of how quality data can empower the best business decisions. Data Centric Inc. covers data quality in a short video, and we've included some of of our own tips below.
1. You have data, but it's not usable yet.
At this point, you just have values in a database or an Excel sheet. This raw data doesn't have a practical use. For instance, you have thousands of email addresses from your customers and their topics of interest in a CSV.
2. You transform data into information.
You take that data to a tool where you can visualize it clearly in the right context. For example, an emailing list inside your marketing app. Now you can filter those email addresses according to their interests.
3. You obtain knowledge.
You analyze the information you've gathered and gain important insights from it. You might learn, for example, that 80% of your customers want to be contacted via email to get information about CRMs.
4. You make an informed decision.
With that knowledge, you can make a data-driven decision, such as deciding to create a newsletter with content about CRMs. When you have quality data, you have the necessary knowledge to make the right decisions for your business.
Characteristics of Data Quality
Since data comes in all shapes and sizes, it's not always easy to determine its quality. However, there are some characteristics typically attributed to high-quality data. Here are six examples of data quality characteristics to look for in your own data.
1. Accuracy
Is your data correct? And does it reflect the context of the situation in which you're using the data?
No matter how much data you manage, if it isn't accurate, it won't be very helpful to your business. Inaccurate data can also challenge your data integrity which exposes your organization, employees, customers, and other stakeholders to unwanted consequences like decreased trust in your business.
To ensure the accuracy of your data, you'll want to employ a good data management strategy that is both sustainable and effective.
2. Completeness
Is your data comprehensive? Incomplete information might be unusable.
Though it's not advisable to collect more than the strictly necessary, make sure your must-have values are mandatory when storing new entries in your database. Otherwise, you'll end up with first names without last names, or incomplete phone numbers you can't use.
3. Relevance
Is this the data you need? Let's face it, not all the data you collect is going to be a game-changer. But if there's a reason why you are collecting data and the values you obtained can serve that purpose, then you have quality data.
For example, if you ask your customers what their birth year is when they're signing up for a trial with your product, but their age is not actually useful information to you, it's data without a purpose. Therefore, even if it's correct, that data isn't relevant to your business's needs. Having unnecessary data in your database can take away valuable time and resources you've dedicated to data security.
4. Consistency
Does your data contradict other sources? High-quality data shouldn't contradict the data stored in other databases. Otherwise, you would have to assume one of them is wrong — but which one?
When there are inconsistencies between databases, it's a hassle to determine accuracy. Instead, ensure there's one source of truth when it comes to your data — whether that means getting everyone on the same data software or integrating your data tool with your CRM.
This way, everyone within your organization can access your data via a single tool, no matter where they are or when they need access.
5. Accessibility
Is the information accessible to the right people? Similar to the previous point we just covered, many companies interact with customers, prospects, partners, and employees via different applications.
As a result, data is scattered throughout different tools, and if there's no software integration in place, you have a data silos problem.
Data silos are among the main causes of poor data quality. Even with accurate, consistent, and relevant data, if the team who should be leveraging that information doesn't have access to it, it's not serving its purpose. To guarantee accessibility, integrate your business systems.
6. Timeliness
Is your data up-to-date? Data is constantly changing, and the problem with outdated data is that it may not be representative of the current situation. It's great to keep track of historical data, but with a clear sense of time.
Ensure you're keeping your data records but you'll also want real-time data and reports so you're aware of any changes as they're happening. This way you can either capitalize on those changes or work to mitigate any issues as needed.
Data Quality Analysis
Data quality analysis is how you ensure your data is... well, high quality.
In other words, it allows you to make sure your data is: accurate, relevant, up-to-date, and suited for its intended use and application.
Data quality analysis is often part of the process of data quality management.
Data Quality Management
Data quality management is the process of ensuring your team has access to high-quality data — it entails pulling insights about the health of your data in order to improve upon that health. This leads to the application of accurate data and the creation of larger data sets.
Data Quality Metrics
Data quality metrics are how you actually determine the quality of your data — they're the unique measurements that you put in place to analyze your data.
Data quality metrics are what actually determine the level of your data's accuracy, relevancy, application, etc. — as a result, you'll know how high (or low) quality your data is.
Here are some of the most common data quality metrics to watch:
- Number of Empty Values
- Number of Duplicate Values
- Data Storage Costs
Data Quality Management Best Practices
Here are some data quality management best practices to keep in mind while analyzing the quality of your data.
1. Determine your team's most important metrics.
You can use the metrics we've outlined above or create new ones. The key here is to choose and track only the metrics that will help you make decisions. For example, you wouldn't want to report on the total number of data entries if your team does not have a goal to attain more data entries.
2. Get data quality buy-in across your business so everyone understands its importance.
Data is one of those behind-the-scenes functions that often gets overlooked. If you're responsible for data quality and management at your organization, you'll have great success with your efforts when you get buy-in from stakeholders across the business. When everyone understands that their success depends on quality data, you'll be more likely to get the resources and support you need to strengthen your data management strategy.
3. Ensure there's a single source of truth across your organization when it comes to your data (whether in your CRM, sales software, etc.).
As we mentioned earlier, if you have several places to store your data and each database has discrepancies, you'll have to choose one to be your source of truth. Deciding which one to choose shouldn't be an arbitrary decision. By performing a data quality audit, you can begin to understand which database is aligned with data quality characteristics we outline at the beginning of this post.
Speaking of performing quality data audits…
4. Perform data quality audits regularly.
The best way to mitigate a problem is to prevent it. Performing regurlar data quality audits can help you spot potential issues before they get bigger. Your audit doesn't have to be complex, simply check on the metrics you outlined earlier in this post. You can do this on a weekly or monthly schedule, or even more often if you manage a lot of data.
5. Dive into the reasons for any data quality failures or notable successes that your team experiences.
Did you team have a great win? Great! Find out why so you can repeat them. Do the same if your team encounters a roadblock. Optimizing your successes and troubleshooting your roadblocks can help your team become more effective at collecting and maintaining quality data.
6. Invest in the necessary resources for data reporting, analysis, and quality training.
Once you have your data management environment set up, it's a good idea to put reporting, analysis, and training systems in place to maintain it. These tools can be acquired separately, or you can use one data quality management tool for all of these functions.
7. Use a data quality management tool.
It's one thing to have data, but it's another to make it easily accessible. One way to do this is to choose the right system for the reporting and analysis of your data. With so many systems to choose from, it can be difficult to pick one, but we've got you covered with the top data quality tools below.
Data Quality Tools
Here are some powerful data quality tools to help you accomplish everything we mentioned above and more.
1. Operations Hub
Operations Hub lets you easily sync customer data and automate business processes. Your team will stay aligned with a clean, connected source of truth for customer data, and your business will be empowered to adapt to the ever-changing needs of your customers.
Operations Hub automates the process of data quality analysis — rather than programmable automation (a.k.a. choose-your-own-adventure), the data quality actions in HubSpot are pre-made and out-of-the-box.
HubSpot's Ops Hub includes three unique programmable automation features: 1) custom coded workflow actions, 2) custom coded bot actions, and 3) webhooks in workflows. Speaking of workflows, you can use them to automate and solve common data issues. For instance, you might set up a workflow that capitalizes the first name property whenever a new contact fills out a demo form.
2. Insycle
Insycle — a HubSpot App Partner and integration — is a complete customer data management solution. It helps you manage, automate, and maintain your customer data. Insycle improves efficiency, reporting accuracy, and team alignment.
3. Dedupely
Dedupely finds and merges duplicate data automatically, saving you time and headaches and improving confidence and alignment across your company.
4. SAS
SAS is an enterprise software suite with products that manage, improve, integrate, and govern your data. One of its best-reviewed products is SAS Data Management — it's designed to manage data integration and cleansing. The tool also provides powerful ways to implement data governance.
SAS also offers SAS Data Quality as a solution to address data quality issues without the need to move your data.
5. Talend Open Studio
Talend Open Studio is part of an open-source suite ideal for mid-market businesses. The drag-and-drop builder makes it flexible and easy to use. Talend comes with several features meant for helping you solve integration problems.
6. OpenRefine
OpenRefine (formerly Google Refine) is a free, open-source tool for businesses of all sizes — it's meant for managing and cleaning data.
OpenRefine focuses on transforming and reformatting disparate data to standardize it. This software allows you to add countless extensions and plugins so you can work with multiple data sources and formats.
7. Datawarehouse.io (Ultimate Data Export)
The Datawarehouse.io, also known as Ultimate Data Export, is a data warehousing middleware solution for your HubSpot data — by syncing the software, your HubSpot platform and data will be integrated without the need for code.
Seamlessly export all of your HubSpot data (e.g. tickets, products, emails, and web analytics) to Excel as well as integrate your data with business intelligence tools, like Tableau.
8. Ataccama
Ataccama is a data management and governance platform with tools for data quality, data management, data catalog, reference data management, data integration, and data profiling.
The tool's data analysis and management features provide insight into the quality of your data. They also help you validate your data, improve upon it, filter out any low-quality or incorrect data, and monitor quality over time.
Get a Comprehensive Overview of Data Quality
Guaranteeing data quality is not always easy, but the time and effort you put into it will pay off in the long-term success of your business. It allows team leaders to make informed and data-driven decisions.
Not everyone can be a data expert, but there are some key concepts, techniques, and tools that make it possible for every professional to improve their data quality.
Editor's note: This post was originally published in October 2020 and has been updated for comprehensiveness.