I still remember the day I launched my first AI-powered chatbot. I must admit that I was totally glued to the screen as we went live, watching its real-time interactions with customers, feeling both excited and a little anxious.
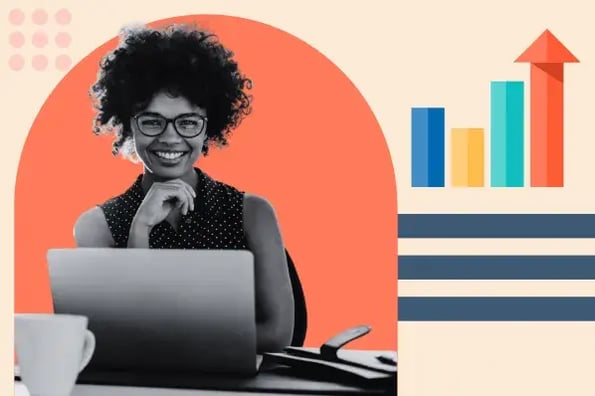
Could the technology actually understand the nuances of human language and provide the kind of support our customers were looking for? Would it improve their overall experience or just make things worse?
The results were surprising. The chatbot was building rapport, resolving issues effectively, and even exceeding customer expectations. It also freed up my team to focus on more complex issues and other project work. Our CSAT scores went up, and we saw an increase in feature adoption for customers coming out of product onboarding. It was an effective example of how AI could transform a business.
Sure, the chatbot was a win. But that was just the beginning of my journey into exploring the world of predicting customer behavior. I thought to myself, if we could use AI tools to predict customer behavior in support, what else could we do? How could we use this technology to create more personalized experiences, anticipate needs, and builder stronger relationships with customers?
In this article, I’ll share what I’ve learned about why understanding your customer is so important, how AI can help you anticipate their needs and actions, and the real-world impact this can have on your business. By the end of this article, you’ll have a solid understanding of how to leverage AI to get inside your customers’ minds and create experiences that continue to WOW them on a regular basis.
Table of Contents
- What Is Customer Behavior?
- Why Predicting and Analyzing Customer Behavior is Important
- The Benefits of Using AI for Customer Behavior Analysis
- How to Predict Customer Behavior Using AI
- How to Analyze Customer Behavior with AI
- The Future is Predictive: Transforming Customer Behavior with AI
.webp)
Free Customer Journey Template
Outline your company's customer journey and experience with these 7 free templates.
- Buyer's Journey Template
- Future State Template
- Day-in-the-Life Template
- And more!
Download Free
All fields are required.
.webp)
What Is Customer Behavior?
So, what is customer behavior anyways? It’s essentially the behaviors, decisions, and preferences people tend to place importance on when engaging with products or services. It can typically consist of things like purchasing patterns, beliefs, and dedication. Customers can be affected by a wide range of forces such as individual tastes, societal expectations, and promotional activities.
Now, understanding those forces and influences is important, but it’s only the first step. You’ll start to notice real transformation when you can predict those behaviors. By anticipating what your customer will do next, you can create proactive experiences that exceed their expectations.
Why Predicting and Analyzing Customer Behavior is Important
In my experience managing ecommerce, I’ve realized that understanding customer behavior is extremely important for creating “sticky” experiences and critical for your business’ success. At the beginning, I depended on intuition and basic data analysis to make decisions. But as business grew, I quickly realized we needed a more sophisticated approach.
It helps you stay ahead of customer needs.
By predicting customer behavior, we can anticipate needs before they even come up. I’ve personally seen how a proactive approach can dramatically improve CSAT scores. For example, at one company, when we started predicting seasonal buying patterns, we were able to stock up on popular items early and place them at the forefront of our ecommerce experience. This led to an increase in holiday sales and a better understanding of our customer base.
I remember during that same holiday season, our AI system predicted a surge in demand for a specific product that we hadn’t considered a bestseller. Trusting the data, we increased our inventory — and the results? It became another top-selling item that season. The experience definitely taught me the value of trusting the data over intuition.
The benefits didn’t stop there either. We actually saw a significant drop in customer support tickets about products being out of stock. By using the data and having the right products on hand, we avoided frustrating customers, allowing the team to really hone in on delivering exceptional service to those who did need support.
It personalizes the customer experience.
Analyzing customer behavior allows you to create those “you just get me!” experiences that customers really love.
I remember when we first started using personalized product recommendations as little popups that would appear as you were shopping (nothing obtrusive, of course). Our average order value increased within the first month — it was actually surprising. And honestly, this is the level of personalization customers are looking for these days.
In fact, a 2023 State of the Connected Customer report by Salesforce found that 73% of customers expect better personalization as technology advances, and 74% expect better personalization when they provide more of their data.
So how can you get started with personalization? Like I said, it all starts with data. You need to gather information about your customers, data like:
- Demographics
- Behavioral characteristics
- Cultural background
- Social experiences
- Economic factors
Once you have this, you can get started with creating targeted campaigns, personalized recommendations, and custom experiences.
It reduces churn and increases loyalty.
Let’s talk about churn, that dreaded word that keeps every business owner up at night. Losing customers is a bummer; there’s no two ways about it. But the good news is that by predicting customer behavior, you can actually get ahead of it.
According to various studies reported in a 2023 SEMrush blog post, retaining an existing customer is significantly easier than acquiring a new one. Businesses have a 60% to 70% chance of selling to an existing customer, while the probability of selling to a new prospect is just 5% to 20%. You can achieve this by implementing proactive measures to identify and engage at-risk customers.
You’ll need to analyze customer data to identify patterns and behaviors that may indicate a customer is considering leaving. This could include things like decreased purchase frequency, reduced website activity, lower product engagement, or negative feedback on surveys, amongst other indicators. Once you identify these at-risk customers, you can then implement targeted retention strategies.
By reducing churn, you can also prioritize customer loyalty. Loyal customers are more likely to make repeat purchases, spend more, and advocate for your brand. One way to do this is by providing exceptional customer service. Providing prompt, helpful, and friendly support builds trust and encourages customers to return.
A 2024 study by Zendesk found that 95% of customers say that trust in a company underpins their likelihood to be loyal, recommend them, make more purchases, and spend more per purchase. By investing in both churn reduction and loyalty-building strategies, you can create a strong foundation for your business to thrive.
The Benefits of Using AI for Customer Behavior Analysis
AI is fundamentally changing how we understand and interact with our customers. It allows us to see patterns, predict behaviors, and personalize experiences we didn’t necessarily think were possible or desired by customers.
Here are a few benefits that make it so powerful.
Uncovering Hidden Patterns
While your team might spend weeks analyzing last quarter’s churn data, AI can process years of historical data in minutes, uncovering patterns invisible to the human eye. Back when I was at Dapper Labs, we were experiencing a season of high support volume. During this time, there was a spike in email tickets related to a specific feature in one of our blockchain-based digital collections.
Using AI, we were able to identify a correlation between the issues and the users who were experiencing them. It allowed our engineering team to priortize fixes for those instances, reducing support tickets and improving the overall collector experience.
Personalizing the Customer Journey
As we uncovered earlier, personalization is no longer a nice-to-have but a customer expectation. AI allows you to deliver personalized experiences at scale, tailoring everything from product recommendations to marketing communication for specific customer segments.
According to a study by DemandSage, companies that provide personlized experiences can generate 40% more revenue than those that don’t. This impact stems from the ability of AI-driven systems to identify customer preferences and deliver the relevant results in real-time.
When I implemented personalized email campaigns at Trendy Butler, a men’s monthly subscription box startup, we saw a significant increase in click-through rates and conversions. By referring to some of the predictive analytics we discovered, we were able to exceed our customers’ expectations, as they appreciated receiving recommendations that were relevant to their style and preferences. As you might expect, this led to higher customer satisfaction and retention.
Predictive Customer Service
Predictive customer service is reshaping how businesses interact with their customers by allowing them to anticipate issues before they even occur. Through the use of AI customer behavior analysis, companies can analyze vast amounts of customer data to identify patterns and trends, which can help predict when and where issues are likely to arise. For example, a customer who frequently checks out but abandons their cart might be flagged for a follow-up message offering assistance. This type of customer behavior prediction can not only help improve cart abandonment rates but also increase customer satisfaction by showing the customer you care about their shopping experience.
The advantages of this approach are clear. It allows businesses to be more responsive and efficient in addressing customer concerns. By predicting problems early, you can resolve them before customers need to reach out. Not to mention, 73% of shoppers believe that AI could improve their customer experience, according to a study done by Tidio.
With customers expecting increasingly seamless and efficient services, predictive support is rapidly becoming a key differentiator.
How to Predict Customer Behavior Using AI
Thanks to AI and machine learning, customer behavior modeling has evolved from a guess to a science. I’ve worked with everything from SaaS companies to ecommerce platforms for ten years, and over that time I’ve seen how these predictive insights can significantly improve customer service, spark growth, and help retain existing customers.
But how do we really go about doing this? Let’s look at a methodical approach to applying AI to customer behavior prediction, supported by concrete data, real-world examples, and industry best practices.
Step 1: Collect and centralize customer data.
The data you collect is the cornerstone of predicting customer behavior. A central system that compiles all customer interactions across touchpoints – including past purchases, browsing patterns, support tickets, social media usage, and even informal information like customer reviews – is important to get started.
In order to centralize data and guarantee that everyone on our team had a single reliable source of truth, at Skybound Entertainment we relied on Shopify’s insights and integrated tools. This made it simple for us to spot trends and irregularities in customer behavior when it came to our store usage.
Pro tip: When you’re collecting customer information, use customer platforms like HubSpot or Segment. According to a KPMG survey, 86% of general U.S. consumers express data privacy as a growing concern for them. It’s important to keep their security at peace.
Step 2: Segment customers based on behavior and demographics.
The next step after centralizing the data is segmentation. Customers can be grouped into meaningful categories by AI according to a variety of characteristics, such as product preferences, frequency of purchases or use, or even social connections. You can find hidden patterns by clustering techniques, such as K-means or DBSCAN.
When I worked at Yahoo, for example, I worked on improving customer segmentation by employing behavior-based segmentation to send targeted ad campaigns to particular groups. Both impression and click-through rates were enhanced by this.
Pro tip: Make use of programs with built-in segmentation capabilities, such as Mixpanel and Google Analytics. Use predictive analytics models to learn how various groups act through their buyer behavior journey.
Step 3: Apply predictive analytics models.
After segmenting your customer data, you’ll then want to start applying predictive models to it. Predictive analytics anticipates future behavior by using historical data. As an example, you can use a customer’s historical behavior to predict when they are likely to churn.
At Trendy Butler, I put in place churn prediction models that identified at-risk subscribers by using data on customer behavior, such as frequency of use and their interactions. We improved customer retention by 20% just by implementing tailored marketing and communications prior to customers unsubscribing.
Pro tip: Make use of AI technology that provides already trained models for predicting customer behavior, such as DataRobot or Tableau. Large datasets can be swiftly analyzed by these tools, which produce useful insights that can motivate proactive efforts.
Step 4: Automate responses based on predictions.
AI makes it possible to automate processes that can result in tailored answers once possible customer behaviors have been identified. In my experience, increasing engagement and decreasing customer friction have been largely done by the use of automated responses.
With the help of predictive insights, I’ve implemented AI-driven processes on Discord and Zendesk for some web3 startups I’ve previously consulted to automatically handle frequent customer complaints, resulting in dramatically improved response times, upwards of 50%.
Pro tip: Use chatbots driven by AI platforms like Intercom, Drift, and one of my personal favorites, Ada CX. These systems guarantee prompt and individualized interaction by having the ability to react to customers on their own, or forward problems to the appropriate team member when necessary.
Step 5: Continuously monitor and optimize predictions.
When it comes to customer behavior prediction, it’s not as easy as “set it and forget it.” It’s an ongoing process that requires constant focus on the little things. To ensure that your plants thrive, you must constantly water, weed, and prune them, much like you would with a garden. Since customer tastes and behaviors are ever-evolving, it’s important to continuously analyze and develop your predictive models in order to stay ahead of the curve.
This is something we heavily emphasized at Dapper Labs when we were building the conversational AI chatbots. We regularly reviewed our predictive models and adjusted them based on real-time customer feedback and real-time customer usage. The results? The chatbots were able to retain an astonishing 70% of inbound volumes, freeing up time and cost for the CX department.
Pro tip: Regularly retrain your AI models using fresh customer data. Tools like H2O.ai or Google Cloud AI offer capabilities to refine models over time, making sure they adapt to new behaviors and trends in your customer base.
Step 6: Use predictive insights for strategic decision-making.
Predictive analytics is transforming how businesses make strategic decisions by enabling them to anticipate customer behavior and take preventative actions. This approach goes beyond straightforward historical data analysis by utilizing AI and machine learning to identify trends and predict outcomes.
A McKinsey report claims that companies that employ predictive analytics can expect to increase revenue by 15% and get a 20% ROI. This provides a data-driven approach to customer communication by optimizing marketing efforts, identifying cross-selling opportunities, and streamlining support operations. In an ecommerce setting, it can help estimate abandoned carts based on user behavior using predictive analytics, allowing for customized interventions like real-time product recommendations or promotions.
Pro tip: Make strategic decisions using predicted insights. Examine how predictive models affect important company indicators like revenue, NPS, and CSAT on a regular basis.
How to Analyze Customer Behavior with AI
After predicting customer behavior using AI, the next step is to analyze the accuracy and impact of those predictions. This type of assessment helps guarantee that the data yields measurable results and improves customer interactions while also helping you to improve predicting customer behavior AI.
Here’s a detailed overview on how to analyze your AI-backed predictions.
Step 1: Evaluate prediction accuracy.
Successful AI models are built on accurate predictions. Comparing expectations with actual customer actions is the first step in evaluating the performance of your models. This can help in finding disparities and improving the model’s accuracy.
Important metrics to track:
- Comparison between prediction and actual: For example, if your model predicted a 30% churn rate but the actual churn was only 25%, you may want to alter the model for subsequent predictions by determining the margin error.
- Precision, recall, F1 score: These metrics measure how successfully your model detects true positives (precision), avoids false negatives (recall), and offsets any false negatives (F1 score).
Pro tip: Revalidate your models on a regular basis with new data. Prediction accuracy can alter in response to even the smallest change in customer behavior. You can make the necessary changes on time if your conduct regular performance reviews.
Back when I worked at Dapper Labs, my team and I regularly blocked off time every week to optimize our predictive models. If you fall behind on this, it can take some time to catch up and maintain the accuracy of your models.
Example: A clothing retailer predicted a 15% increase in return rates based on customer behavior, but after analyzing actual return data, they found only 10%. By adjusting their customer behavior prediction and adding more accurate data, their future predictions improved by 20%, leading to better product recommendations.
Step 2: Segment predictions based on customer groups.
Okay, so now we have our predictive models up and running. The problem is that those predictions won’t all be equally correct. As the word suggests, different customer segments are different! They exhibit unique habits, and therefore, segmenting your customer predictions according to demographics, past purchases, or involvement level is very important. You’ll be able to see your customer base more clearly with this granular approach.
Pro tip: Make use of dynamic segmentation, which changes as customer behavior changes. More precise and tailored insights will be provided by predictive models that can adjust over time, particularly in segments where consumer behavior patterns shift more quickly.
Example: A subscription service tested prediction models for different customer segments, such as those with varying degrees of engagement. They discovered that while low-engagement customers required changes to the AI model, leading to more focused engagement tactics, predictions for high-engagement customers ended up being more accurate. It reinforces the importance of a more nuanced approach to predicting customer behavior, one that you can leverage AI to create more personalized and effective strategies.
Step 3: Measure the impact on business outcomes.
The goal of analyzing customer behavior predictions is to have a positive impact on your business. Evaluate how accurate your predictions are influencing business metrics like conversion rates, customer lifetime value, and retention.
Key metrics to measure:
- Conversion rate improvement: If your model predicted customers likely to convert after a specific interaction or event, compare the actual conversions against the predicted ones.
- Customer lifetime value (CLV): For retention predictions, track the CLV of predicted “loyal” customers versus those predicted to churn but ended up staying.
Pro tip: I like to directly correlate the measurement of the predictive models to the success of the business KPIs. If a model is improving customer retention or increasing conversions, track how these improvements affect your bottom line. Then, use these insights to make data-backed decisions for scaling your AI-driven initiatives.
Example: After implementing predictive analytics to forecast customer churn, a SaaS company noticed a 25% increase in their retention rates by proactively addressing at-risk customers with tailored outreach. This led to higher CSAT scores and a boost in CLV.
Step 4: Refine predictions with real-time data.
AI models should be continuously retrained with real-time data to keep them relevant and accurate. Real-time data integration allows the AI systems to learn from the latest customer interactions and trends available to you, improving the accuracy of future predictions.
Pro tip: I like to use data enrichment tools to add external sources, attributes like social media activity or third-party behavioral data, to my real-time data streams. This can offer a more holistic view of customer preferences.
Example: An online marketplace integrated real-time browsing data to fine-tune predictions for their product recommendations. They saw a 40% improvement in recommendation accuracy and a 15% spike in sales. These refinements also allowed them to offer more timely and personalized promotions.
Step 5: Test and validate with A/B testing.
A/B testing is one of the most effective methods for verifying AI-driven predictions. It enables you to assess the effect on customer behavior by comparing a test group (with predictive inputs) to a control group (without predictive inputs). This allows for faster hypothesis generation and more targeted variable selection, making A/B testing smarter and less resource-intensive.
Pro tip: Always test on multiple segments to ensure that your model works across different customer types. A/B testing helps not only validate predictions but also to discover areas where the model may need further optimization.
Example: A fintech company tested predictive models for cross-selling financial products by segmenting users into A/B test groups. The group receiving the predictions tailored to their financial goals saw a 12% higher conversion rate compared to the control group.
Step 6: Continuously optimize with customer feedback.
Customer feedback is an important resource for improving predictive models since it provides information about how well AI predictions match user expectations. Finding differences between expectations and real-world outcomes can be improved by collecting qualitative data through surveys, NPS, or direct feedback from customers.
Pro tip: I personally like sentiment analysis tools like Brandwatch, MonkeyLearn i.e. Medallia, and HubSpot’s Service Hub to evaluate feedback and gauge how customers feel about the recommendations they receive. This can help identify whether your predictions are over- or under-delivering.
Example: A store modified product recommendations based on real-time customer input following the launch of a new recommendation engine. By more closely matching product recommendations to consumer preferences, they increased satisfaction by 18%.
The Future is Predictive: Transforming Customer Behavior with AI
Understanding AI customer behavior analysis is a dynamic, continuous process that calls for constant optimization and close attention to detail. Businesses can improve their models and make sure they’re getting the most out of their insights by measuring predictive accuracy, dividing up predictions by customer groups, calculating their impact on business outcomes, and integrating real-time data and customer feedback.
In addition to increasing prediction precision, this iterative process improves customer satisfaction and produces better business outcomes for all parties.
I’ve come to realize that evaluating the accuracy of predictions is one of the important aspects of improving AI models. In my own work, I’ve seen how adjusting AI models based on precision, recall, and F1 scores can make a difference in predicting customer behaviors like churn or engagement. It’s not just about numbers, it’s about refining the model to align more closely with actual customer behavior. The importance of using precision and recall metrics is something I’ve found valuable when evaluating prediction models, ensuring that businesses are always on target and can make proactive adjustments.
Overall, I’ve further realized that the real value in AI customer behavior analysis comes from the ability to constantly optimize. This ongoing effort has allowed me to refine my own strategies over the years, leading to improved customer satisfaction and stronger business outcomes. By combining predictive models with real-time data, feedback, and A/B testing, businesses can maximize their AI-driven efforts, fostering customer experiences that are both personalized and optimized for success.
.webp)
Free Customer Journey Template
Outline your company's customer journey and experience with these 7 free templates.
- Buyer's Journey Template
- Future State Template
- Day-in-the-Life Template
- And more!
Download Free
All fields are required.
.webp)