Obtaining customer feedback is difficult. You need strong survey questions that effectively derive customer insights. Not to mention a distribution system that shares the survey with the right customers at the right time.However, survey data doesn't just sort and analyze itself. You need a team dedicated to sifting through survey results and highlighting key trends and behaviors for your marketing, sales, and customer service teams. In this post, we'll discuss not only how to analyze survey results, but also how to present your findings to the rest of your organization.
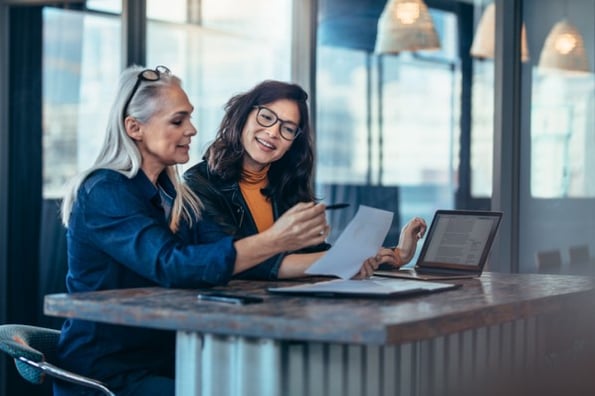
Short on time? Jump to the topics that interest you most:
Survey Report Template Examples
How to Analyze Survey Results
1. Understand the four measurement levels.
Before analyzing data, you should understand the four levels of measurement. These levels determine how survey questions should be measured and what statistical analysis should be performed. The four measurement levels are nominal scales, ordinal scales, interval scales, and ratio scales.
Nominal Scale
Nominal scales classify data without any quantitative value, similar to labels. An example of a nominal scale is, "Select your car's brand from the list below." The choices have no relationship to each other. Due to the lack of numerical significance, you can only keep track of how many respondents chose each option and which option was selected the most.
.png)
Free Market Research Kit
5 Research and Planning Templates + a Free Guide on How to Use Them in Your Market Research
- SWOT Analysis Template
- Survey Template
- Focus Group Template
- And More!
Download Free
All fields are required.
.png)
Ordinal Scale
Ordinal scales are used to depict the order of values. For this scale, there's a quantitative value because one rank is higher than another. An example of an ordinal scale is, "Rank the reasons for using your laptop." You can analyze both mode and median from this type of scale, and ordinal scales can be analyzed through cross-tabulation analysis.
Interval Scale
Interval scales depict both the order and difference between values. These scales have quantitative value because data intervals remain equivalent along the scale, but there's no true zero point. An example of an interval scale is in an IQ test. You can analyze mode, median, and mean from this type of scale and analyze the data through ANOVA, t-tests, and correlation analyses. ANOVA tests the significance of survey results, while t-tests and correlation analyses determine if datasets are related.
Ratio Scale
Ratio scales depict the order and difference between values, but unlike interval scales, they do have a true zero point. With ratio scales, there's quantitative value because the absence of an attribute can still provide information. For example, a ratio scale could be, "Select the average amount of money you spend online shopping." You can analyze mode, median, and mean with this type of scale and ratio scales can be analyzed through t-tests, ANOVA, and correlation analyses as well.
2. Select your survey question(s).
Once you understand how survey questions are analyzed, you should take note of the overarching survey question(s) that you're trying to solve. Perhaps, it's "How do respondents rate our brand?"
Then, look at survey questions that answer this research question, such as "How likely are you to recommend our brand to others?" Segmenting your survey questions will isolate data that are relevant to your goals.
Additionally, it's important to ask both close-ended and open-ended questions.
Close-Ended Questions
A close-ended survey question gives a limited set of answers. Respondents can't explain their answer and they can only choose from pre-determined options. These questions could be yes or no, multiple-choice, checkboxes, dropdown, or a scale question. Asking a variety of questions is important to get the best data.
Open-Ended Questions
An open-ended survey question will ask the respondent to explain their opinion. For example, in an NPS survey, you'll ask how likely a customer is to recommend your brand. After that, you might consider asking customers to explain their choice. This could be something like "Why or why wouldn't you recommend our product to your friends/family?"
3. Analyze quantitative data first.
Quantitative data is valuable because it uses statistics to draw conclusions. While qualitative data can bring more interesting insights about a topic, this information is subjective, making it harder to analyze. Quantitative data, however, comes from close-ended questions which can be converted into a numeric value. Once data is quantified, it's much easier to compare results and identify trends in customer behavior.
It's best to start with quantitative data when performing a survey analysis. That's because quantitative data can help you better understand your qualitative data. For example, if 60% of customers say they're unhappy with your product, you can focus your attention on negative reviews about user experience. This can help you identify roadblocks in the customer journey and correct any pain points that are causing churn.
4. Use cross-tabulation to better understand your target audience.
If you analyze all of your responses in one group, it isn't entirely effective for gaining accurate information. Respondents who aren't your ideal customers can overrun your data and skew survey results. Instead, if segment responses using cross-tabulation, you can analyze how your target audience responded to your questions.
Split Up Data by Demographics
Cross-tabulation records the relationships between variables. It compares two sets of data within one chart. This reveals specific insights based on your participants' responses to different questions. For example, you may be curious about customer advocacy among your customers based in Boston, MA. You can use cross-tabulation to see how many respondents said they were from Boston and said they would recommend your brand.
By pulling multiple variables into one chart, we can narrow down survey results to a specific group of responses. That way, you know your data is only considering your target audience.
Below is an example of a cross-tabulation chart. It records respondents' favorite baseball teams and what city they reside in.
5. Understand the statistical significance of the data.
As we mentioned in the last section, not all data is as reliable as you may hope. Everything is relative, and it's important to be sure that your respondents accurately represent your target audience.
For instance, your data states that 33% of respondents would recommend your brand to others. 75% of them were over 40 years old, yet your target audience is 18 to 29 years old. In this case, this data isn't statistically significant as the people who took your survey don't represent your ideal consumer.
.png)
Free Market Research Kit
5 Research and Planning Templates + a Free Guide on How to Use Them in Your Market Research
- SWOT Analysis Template
- Survey Template
- Focus Group Template
- And More!
Download Free
All fields are required.
.png)
Random sampling — selecting an arbitrary group of individuals from a larger population — can help create a more diverse sample of survey responses. Additionally, the more people you survey, the more accurate the results will be.
When you run an analysis on software like SPSS — as shown above — it will tell you if a data point is statistically significant using a p-value. If you look just below the table, it says "*. Correlation is significant at the 0.05 level (2-tailed). **. Correlation is significant at the 0.01 level (2-tailed)." This indicates which values are statistically relevant in your analysis.
If the statistical significance or p-value for a data point is equal to or lower than 0.05, it has moderate statistical significance since the probability for error is less than 5%. If the p-value is lower than 0.01, that means it has high statistical significance because the probability for error is less than 1%.
6. Consider causation versus correlation.
Another important aspect of survey analysis is knowing whether the conclusions you're drawing are accurate. For instance, let's say we observed a correlation between ice cream sales and car thefts in Boston. Over a month, as ice cream sales increased so did reports of stolen cars. While this data may suggest a link between these variables, we know that there's probably no relationship.
Just because the two are correlated doesn't mean one causes the other. In cases like these, there's typically a third variable — the independent variable — that influences the two dependent variables. In this case, it's temperature. As the temperature increases, more people buy ice cream. Additionally, more people leave their homes and go out, which leads to more opportunities for crime.
While this is an extreme example, you never want to draw a conclusion that's inaccurate or insufficient. Analyze all the data before assuming what influences a customer to think, feel, or act a certain way.
7. Compare new data with past data.
While current data is good for keeping you updated, it should be compared to data you've collected in the past. If you know 33% of respondents said they would recommend your brand, is that better or worse than last year? How about last quarter?
If this is your first year analyzing data, make these results the benchmark for your next analysis. Compare future results to this record and track changes over quarters, months, years, or whatever interval you prefer. You can even track data for specific subgroups to see if their experiences improve with your initiatives.
Now that you've gathered and analyzed all of your data, the next step is to share it with coworkers, customers, and other stakeholders. However, presentation is key in helping others understand the insights you're trying to explain.
The next section will explain how to present your survey results and share important customer data with the rest of your organization.
How to Present Survey Results
1. Use a graph or chart.
Graphs and charts are visually appealing ways to share data. Not only are the colors and patterns easy on the eyes, but data is often easier to understand when shared through a visual medium. However, it's important to choose a graph that highlights your results in a relevant way.
This is an example of a stacked bar graph my team created using data on the brand Allbirds. If you're having trouble reading it, that's okay! We received feedback that it was confusing to understand. That's because the data wasn't organized in a way that would make sense to a stakeholder who's unfamiliar with our project.
So, we decided to revamp our graph's image and came up with the design below.
This bar graph is much simpler to read because it has individual bars for each variable and a clear key. And, the design fits the data that we're trying to display. A reader can easily understand the information we obtained from our survey.
Depending on the survey you've conducted, there are many types of graphs and charts you can use. A few options you can choose from are pie charts, Venn diagrams, line graphs, scatter plots, histograms, pictograms, and more. Be sure to pick one that accurately displays your data and is clear to your stakeholders.
2. Create a data table.
Tables are a great way to share numerical data. You can use software like SPSS or Excel to easily display data, like in the example below.
This table was created from a cross-tabulation analysis. We removed the unnecessary information — statistical significance, mean, median, etc. — and focused on the data we wanted to share: the percentage of each gender that preferred each promotional incentive. This gave us a format that demonstrated the percentages we were looking to share with our stakeholders.
3. Tell a story with data analysis.
Vice president of merchandising at Chewy, Andreas von der Heydt, shared a profound message on LinkedIn about the power of storytelling with data. In the image below, Legos are arranged in five different ways: a random assortment, color-coded, arranged in varying heights, positioning in a visually appealing way, and constructed into a house.
One of the primary goals of good data analysis is to weave information together so that it builds upon each other — just like building a house. Some data will serve as the foundation of your story, all the points in your presentation will tie back to this foundation. From there, you'll structure your key findings like the walls of a house. These key findings support the conclusion of your research which acts as the roof. That's the primary point you want to make when presenting your data analysis.
Communicating data can be tricky when your stakeholders have varying degrees of analytical savviness. But no matter how sophisticated (or not) your team is, a story will resonate no matter what. So take the time to identify the point the data leads to and structure a story around that conclusion.
.png)
Free Market Research Kit
5 Research and Planning Templates + a Free Guide on How to Use Them in Your Market Research
- SWOT Analysis Template
- Survey Template
- Focus Group Template
- And More!
Download Free
All fields are required.
.png)
4. Make a visual presentation.
Sometimes combining visuals with text creates a thorough description of your findings. In these cases, a presentation could be a good fit for showcasing your data. This gives you a chance to present the earlier stages of your survey, including research questions, hypotheses, survey questions, and methods of analysis.
This slide from my presentation combines a graph with a table and some text. The same data is shared in three different ways to appeal to people with different learning styles: those who prefer visuals, those who prefer numbers, and those who prefer words.
5. Put together an infographic.
If you need to share data that's easy to read and quickly consumed, infographics might be your best bet.
This HubSpot Research infographic explains survey results through icons, numbers, and descriptive text. Infographics are incredibly effective for this purpose, breaking down complex ideas into simple messages that are more appealing to read than blocks of text.
Using Survey Results in a Report
Sometimes those blocks of text are essential for persuading stakeholders. If you're presenting data to senior executives or business clients, you might want to prepare a full report on your findings. You wouldn't refer to this document during a presentation, but you might hand this to your audience to read through on their own time.
This is the table of contents page from my report on our survey project. It's important to keep track of all the work you've done and maintain records of how you conducted your survey. That way, you won't make similar errors or have to duplicate any research.
How to Write a Survey Report
- Decide the outcome of the survey.
- Write your research summary.
- Create an outline for the report.
- Choose a layout.
- Include the methodology of your research.
- Mention any limitations in your research.
- Add appendices if needed.
1. Decide the outcome of the survey.
Believe it or not, writing the intro to a survey report isn't always the best first step. To keep your report focused on a specific outcome that you want the reader to take away, start by explaining the outcome in detail. This section of the survey report will be included in the middle, but it's a great way to get your bearings when writing — especially with a longer report.
The outcome of the survey report should reveal what could happen if the stakeholders reading the report were onboard with your analysis. Some ideas for outcomes could be a new product offering as a result of customer feedback, adjusting the price of a service to increase revenue, or entering into a new market that shows potential for the organization to expand.
When writing this section, be sure that the data you've collected supports the outcome fully. It's best to avoid ideas that can't be substantiated by the other information in the report.
2. Write your research summary.
Next, summarize your research findings. In this part of the survey report, you'll include notable results and any information that correlates with other studies your company may have done in the past. This summary will be the part of your survey report that people will focus on because it's a condensed version of all the details you've discussed elsewhere in the report.
The research summary should be no more than a page long. To make it even easier to read, include headers above paragraphs to guide the reader through the content.
3. Create an outline for the report.
Now that you have your outcome and summary, it's time to develop the outline. Because the survey report is typically around eight to ten pages long, you'll want to use a concise outline that includes all the relevant information the stakeholders will want to know. Here's a sample outline you can customize:
- Introduction - setting the stage for the report.
- Summary - summarizing the findings and key elements of the research.
- Methodology - explaining how the research was conducted.
- Results - describing what the research revealed.
- Outcomes - analyzing the results in the context of your organization's goals.
- Limitations - revealing any research oversights or areas for further research.
- Conclusion - a summary of the research analysis and why the audience should care about it.
4. Choose a layout.
After the outline is complete, you'll have an idea of how much space you'll need for each section. Survey reports can be published in portrait or landscape layouts depending on your preference.
When to use portrait layout for a survey report
Portrait landscape is best used when the report is text-heavy. Charts and graphs with more rows than columns also work well in portrait-style layouts. Avoid dedicating entire pages to images or infographics in this layout as it takes away space from important text-based content.
When to use landscape layout for a survey report
Use a landscape layout for your survey report when your report will be design-heavy. High-quality imagery, graphs, and charts work well in landscape because there's more space for text-based content to the side of the visuals. Too many text-heavy pages can make a landscape report feel much longer than it actually is.
.png)
Free Market Research Kit
5 Research and Planning Templates + a Free Guide on How to Use Them in Your Market Research
- SWOT Analysis Template
- Survey Template
- Focus Group Template
- And More!
Download Free
All fields are required.
.png)
5. Include the methodology of your research.
The methodology section of your report should explain exactly how your survey was conducted, who was invited to participate, and the types of tests used to analyze the data. You might use charts or graphs to help communicate this data.
It's OK to be detailed here — the readers will want to know that the outcomes of the survey are valid and based on relevant research methods. Include the number of participants, how they were selected, and any demographic data you collected about them. Also, include your process for selecting the survey questions and why you chose certain tests for the analyses.
6. Mention any limitations in your research.
No matter how much you prepare before conducting your survey, you'll find some information in the results that could've been more conclusive had you considered another variable. The great part about research is that it can be continued in the future. The limitations section sets the stage for future researchers to pick up where you left off or correct any mistakes you made in the current survey.
7. Add appendices if needed.
If you're fortunate enough that all of the data you present fits neatly into a chart within the report, you may not require an appendix at the end of your report. But if the graphs and charts you include on the pages are truncated versions of large data sets that provide context, you should include them at the end of your report in their raw forms. Throughout the report, reference the appendix so that the reader can review it for a deeper understanding of your content.
Survey Report Template Examples
Canva has free report templates that can be used for survey reports. When choosing a template, look for ones that include placeholders for graphs and images as well multiple page layouts so you have some variety. Below are some of our favorite survey report template examples from Canva.
1. Geometrical Corporate General Report
Not only is this report eye-catching, it includes a table of contents to help organize the report as well as customizable charts and graphs. This template can be customized to include every element in the survey report outline with ease. As a portrait-style layout, you'll love this Canva template for text-heavy analyses and reports.
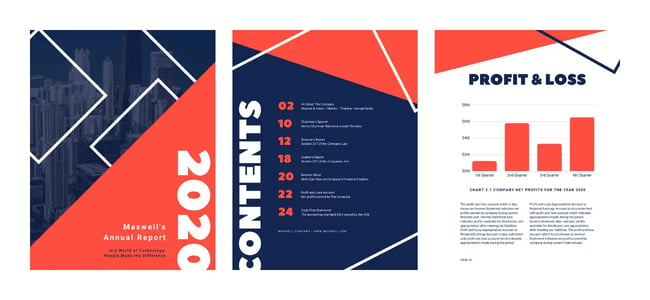
2. Minimal Formal Annual Report
This Canva report template lets the data speak for itself. The minimal portrait layout offers plenty of negative space around the content so that it can breathe. Bold numbers and percentages can remain or be omitted depending on the needs you have for each page. One of the rare gems of this template is its ability to balance large, clear images that don't crowd out the important written information on the page. Use this template for hybrid text-visual designs.
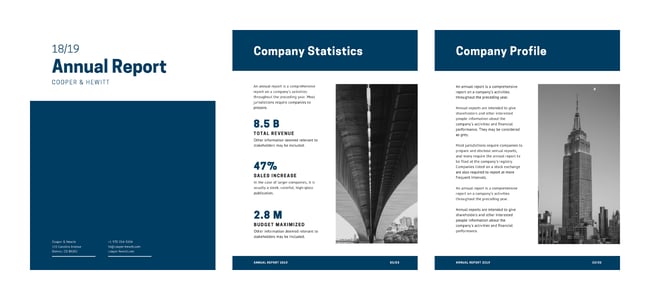
3. Digital Advertising Approach Presentation
Bold and punchy, the Digital Advertising Approach Template by Canva is a landscape-style presentation that can also be used for survey reports. It comes with several types of charts and graphs that you can tailor to your research and data. With more than 28 individual page templates included in this design, you'll probably run out of content in your report before you run out of ways to design it.
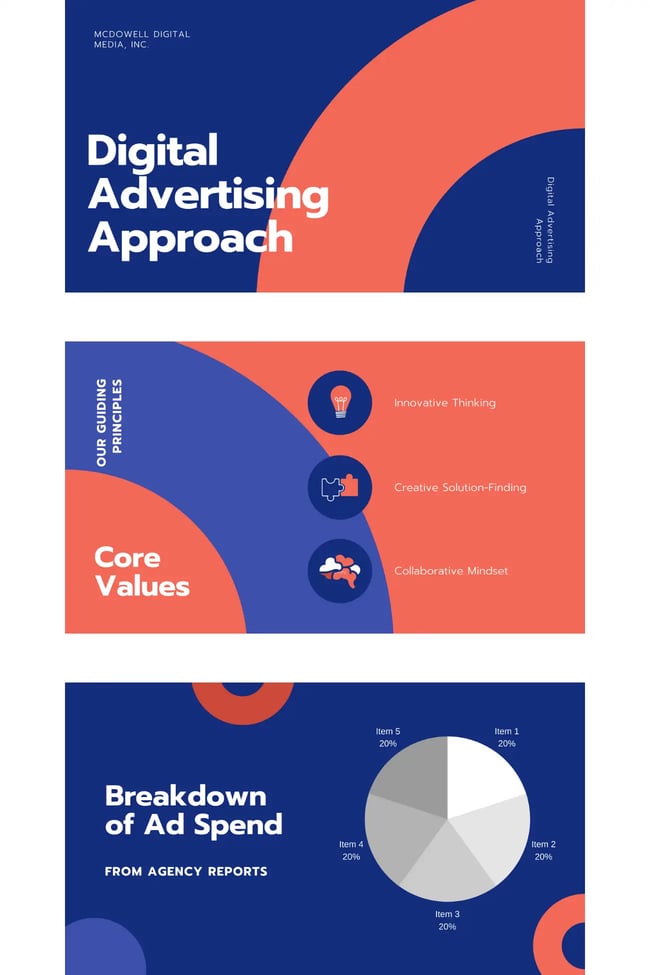
4. Empowerment Keynote Presentation
This presentation template makes a great research report template due to its clean lines, contrasting graphic elements, and ample room for visuals. The headers in this template virtually jump off the page to grab the readers' attention. There's aren't many ways to present quantitative data using this template example, but it works well for qualitative survey reports like focus groups or product design studies where original images will be discussed.
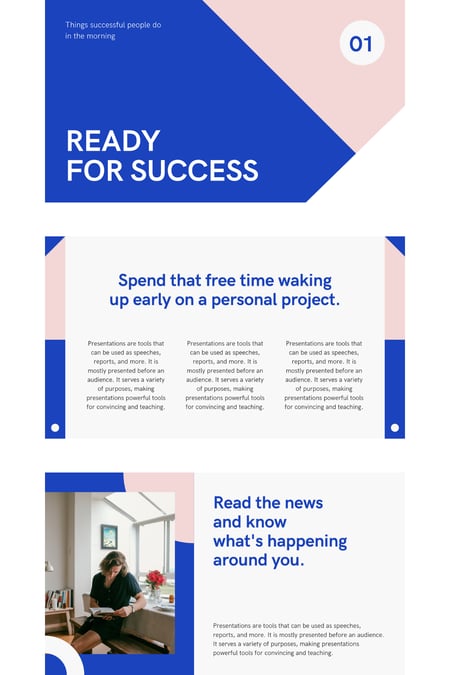
Analyze Survey Data Like A Pro
It's one thing to collect data, but without a strategic analysis process, you could struggle to reach conclusions that justify pursuing your business goals. Follow the tips in this post to produce well-crafted data analyses that will support even the most aspirational projects.
Editor's note: This post was originally published in July 2019 and has been updated for comprehensiveness.