Do you remember your first A/B test you ran? I do. (Nerdy, I know.)
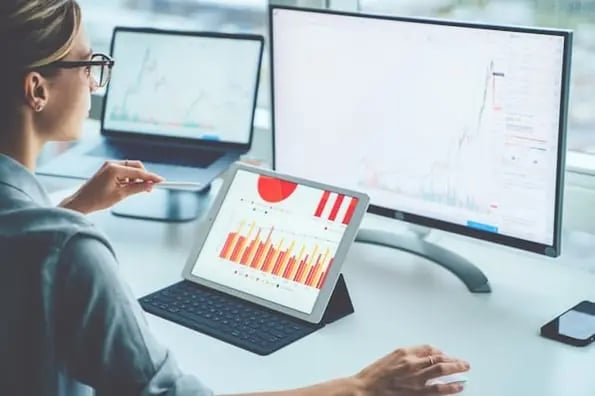
I felt simultaneously thrilled and terrified because I knew I had to actually use some of what I learned in college for my job.
There were some aspects of A/B testing I still remembered — for instance, I knew you need a big enough sample size to run the test on, and you need to run the test long enough to get statistically significant results.
But ... that's pretty much it. I wasn't sure how big was "big enough" for sample sizes and how long was "long enough" for test durations — and Googling it gave me a variety of answers my college statistics courses definitely didn't prepare me for.
Turns out I wasn't alone: Those are two of the most common A/B testing questions we get from customers. And the reason the typical answers from a Google search aren't that helpful is because they're talking about A/B testing in an ideal, theoretical, non-marketing world.
So, I figured I'd do the research to help answer this question for you in a practical way. At the end of this post, you should be able to know how to determine the right sample size and time frame for your next A/B test. Let's dive in.
(Psst: Need to review A/B testing key principles first? This video will help.)
.png)
The Complete A/B Testing Kit for Marketers
Start improving your website performance with these free templates.
- Guidelines for effective A/B testing
- Running split tests for email, landing pages, and CTAs
- Free simple significance calculator
- Free A/B test tracking template.
A/B Testing Sample Size & Time Frame
In theory, to determine a winner between Variation A and Variation B, you need to wait until you have enough results to see if there is a statistically significant difference between the two.
Depending on your company, sample size, and how you execute the A/B test, getting statistically significant results could happen in hours or days or weeks — and you've just got to stick it out until you get those results. In theory, you should not restrict the time in which you're gathering results.
For many A/B tests, waiting is no problem. Testing headline copy on a landing page? It's cool to wait a month for results. Same goes with blog CTA creative — you'd be going for the long-term lead generation play, anyway.
But certain aspects of marketing demand shorter timelines when it comes to A/B testing. Take email as an example. With email, waiting for an A/B test to conclude can be a problem, for several practical reasons:
1. Each email send has a finite audience.
Unlike a landing page (where you can continue to gather new audience members over time), once you send an email A/B test off, that's it — you can't "add" more people to that A/B test. So you've got to figure out how squeeze the most juice out of your emails.
This will usually require you to send an A/B test to the smallest portion of your list needed to get statistically significant results, pick a winner, and then send the winning variation on to the rest of the list.
2. Running an email marketing program means you're juggling at least a few email sends per week. (In reality, probably way more than that.)
If you spend too much time collecting results, you could miss out on sending your next email -- which could have worse effects than if you sent a non-statistically-significant winner email on to one segment of your database.
3. Email sends are often designed to be timely.
Your marketing emails are optimized to deliver at a certain time of day, whether your emails are supporting the timing of a new campaign launch and/or landing in your recipient's inboxes at a time they'd love to receive it. So if you wait for your email to be fully statistically significant, you might miss out on being timely and relevant — which could defeat the purpose of your email send in the first place.
That's why email A/B testing programs have a "timing" setting built in: At the end of that time frame, if neither result is statistically significant, one variation (which you choose ahead of time) will be sent to the rest of your list. That way, you can still run A/B tests in email, but you can also work around your email marketing scheduling demands and ensure people are always getting timely content.
So to run A/B tests in email while still optimizing your sends for the best results, you've got to take both sample size and timing into account.
Next up — how to actually figure out your sample size and timing using data.
How to Determine Sample Size for an A/B Test
Now, let's dive into how to actually calculate the sample size and timing you need for your next A/B test.
For our purposes, we're going to use email as our example to demonstrate how you'll determine sample size and timing for an A/B test. However, it's important to note — the steps in this list can be used for any A/B test, not just email.
Let's dive in.
Like mentioned above, each A/B test you send can only be sent to a finite audience -- so you need to figure out how to maximize the results from that A/B test. To do that, you need to figure out the smallest portion of your total list needed to get statistically significant results. Here's how you calculate it.
1. Assess whether you have enough contacts in your list to A/B test a sample in the first place.
To A/B test a sample of your list, you need to have a decently large list size — at least 1,000 contacts. If you have fewer than that in your list, the proportion of your list that you need to A/B test to get statistically significant results gets larger and larger.
For example, to get statistically significant results from a small list, you might have to test 85% or 95% of your list. And the results of the people on your list who haven't been tested yet will be so small that you might as well have just sent half of your list one email version, and the other half another, and then measured the difference.
Your results might not be statistically significant at the end of it all, but at least you're gathering learnings while you grow your lists to have more than 1,000 contacts. (If you want more tips on growing your email list so you can hit that 1,000 contact threshold, check out this blog post.)
Note for HubSpot customers: 1,000 contacts is also our benchmark for running A/B tests on samples of email sends — if you have fewer than 1,000 contacts in your selected list, the A version of your test will automatically be sent to half of your list and the B will be sent to the other half.
.png)
The Complete A/B Testing Kit for Marketers
Start improving your website performance with these free templates.
- Guidelines for effective A/B testing
- Running split tests for email, landing pages, and CTAs
- Free simple significance calculator
- Free A/B test tracking template.
2. Use a sample size calculator.
Next, you'll want to find a sample size calculator — HubSpot's A/B Testing Kit offers a good, free sample size calculator.
Here's what it looks like when you download it:
3. Put in your email's Confidence Level, Confidence Interval, and Population into the tool.
Yep, that's a lot of statistics jargon. Here's what these terms translate to in your email:
Population: Your sample represents a larger group of people. This larger group is called your population.
In email, your population is the typical number of people in your list who get emails delivered to them — not the number of people you sent emails to. To calculate population, I'd look at the past three to five emails you've sent to this list, and average the total number of delivered emails. (Use the average when calculating sample size, as the total number of delivered emails will fluctuate.)
Confidence Interval: You might have heard this called "margin of error." Lots of surveys use this, including political polls. This is the range of results you can expect this A/B test to explain once it's run with the full population.
For example, in your emails, if you have an interval of 5, and 60% of your sample opens your Variation, you can be sure that between 55% (60 minus 5) and 65% (60 plus 5) would have also opened that email. The bigger the interval you choose, the more certain you can be that the populations true actions have been accounted for in that interval. At the same time, large intervals will give you less definitive results. It's a trade-off you'll have to make in your emails.
For our purposes, it's not worth getting too caught up in confidence intervals. When you're just getting started with A/B tests, I'd recommend choosing a smaller interval (ex: around 5).
Confidence Level: This tells you how sure you can be that your sample results lie within the above confidence interval. The lower the percentage, the less sure you can be about the results. The higher the percentage, the more people you'll need in your sample, too.
Note for HubSpot customers: The HubSpot Email A/B tool automatically uses the 85% confidence level to determine a winner. Since that option isn't available in this tool, I'd suggest choosing 95%.
Email A/B Test Example:
Let's pretend we're sending our first A/B test. Our list has 1,000 people in it and has a 95% deliverability rate. We want to be 95% confident our winning email metrics fall within a 5-point interval of our population metrics.
Here's what we'd put in the tool:
- Population: 950
- Confidence Level: 95%
- Confidence Interval: 5
4. Click "Calculate" and your sample size will spit out.
Ta-da! The calculator will spit out your sample size.
In our example, our sample size is: 274.
This is the size one your variations needs to be. So for your email send, if you have one control and one variation, you'll need to double this number. If you had a control and two variations, you'd triple it. (And so on.)
5. Depending on your email program, you may need to calculate the sample size's percentage of the whole email.
HubSpot customers, I'm looking at you for this section. When you're running an email A/B test, you'll need to select the percentage of contacts to send the list to — not just the raw sample size.
To do that, you need to divide the number in your sample by the total number of contacts in your list. Here's what that math looks like, using the example numbers above:
274 / 1,000 = 27.4%
This means that each sample (both your control AND your variation) needs to be sent to 27-28% of your audience — in other words, roughly a total of 55% of your total list.
And that's it! You should be ready to select your sending time.
How to Choose the Right Timeframe for Your A/B Test
Again, for figuring out the right timeframe for your A/B test, we'll use the example of email sends – but this information should still apply regardless of the type of A/B test you're conducting.
However, your timeframe will vary depending on your business' goals, as well. If you'd like to design a new landing page by Q2 2021 and it's Q4 2020, you'll likely want to finish your A/B test by January or February so you can use those results to build the winning page.
But, for our purposes, let's return to the email send example: You have to figure out how long to run your email A/B test before sending a (winning) version on to the rest of your list.
.png)
The Complete A/B Testing Kit for Marketers
Start improving your website performance with these free templates.
- Guidelines for effective A/B testing
- Running split tests for email, landing pages, and CTAs
- Free simple significance calculator
- Free A/B test tracking template.
Figuring out the timing aspect is a little less statistically driven, but you should definitely use past data to help you make better decisions. Here's how you can do that.
If you don't have timing restrictions on when to send the winning email to the rest of the list, head over to your analytics.
Figure out when your email opens/clicks (or whatever your success metrics are) starts to drop off. Look your past email sends to figure this out.
For example, what percentage of total clicks did you get in your first day? If you found that you get 70% of your clicks in the first 24 hours, and then 5% each day after that, it'd make sense to cap your email A/B testing timing window for 24 hours because it wouldn't be worth delaying your results just to gather a little bit of extra data.
In this scenario, you would probably want to keep your timing window to 24 hours, and at the end of 24 hours, your email program should let you know if they can determine a statistically significant winner.
Then, it's up to you what to do next. If you have a large enough sample size and found a statistically significant winner at the end of the testing time frame, many email marketing programs will automatically and immediately send the winning variation.
If you have a large enough sample size and there's no statistically significant winner at the end of the testing time frame, email marketing tools might also allow you to automatically send a variation of your choice.
If you have a smaller sample size or are running a 50/50 A/B test, when to send the next email based on the initial email's results is entirely up to you.
If you have time restrictions on when to send the winning email to the rest of the list, figure out how late you can send the winner without it being untimely or affecting other email sends.
For example, if you've sent an email out at 3 p.m. EST for a flash sale that ends at midnight EST, you wouldn't want to determine an A/B test winner at 11 p.m. Instead, you'd want to send the email closer to 6 or 7 p.m. — that'll give the people not involved in the A/B test enough time to act on your email.
And that's pretty much it, folks. After doing these calculations and examining your data, you should be in a much better state to conduct successful A/B tests — ones that are statistically valid and help you move the needle on your goals.