Over the last few years, I've researched and written about AI for HubSpot — a lot. At this point in my journey, I'm pretty much obsessed with Informatica's annual survey of global data leaders. As I revisit this research today, it reveals a whopping 100% of the respondents plan to invest in data management capabilities in 2024.
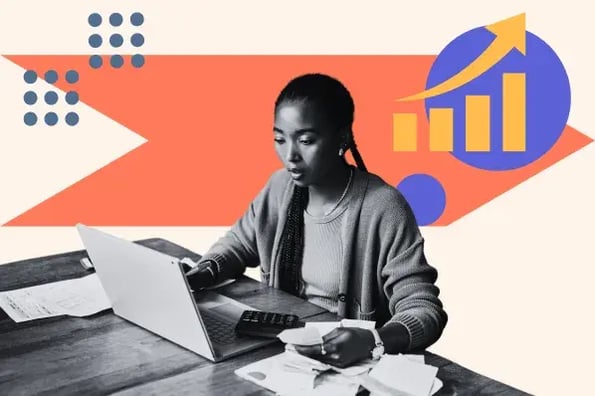
Data leaders see these capabilities as a way to overcome roadblocks to AI adoption within their organizations. But as AI data management is on the rise, I wanted to find out one thing: Exactly how are businesses “leveraging” (sorry!) AI across the different stages of the data lifecycle?
Spoiler alert: I found out! So join me as I “delve” (sorry, sorry!) into the topic at hand, covering:
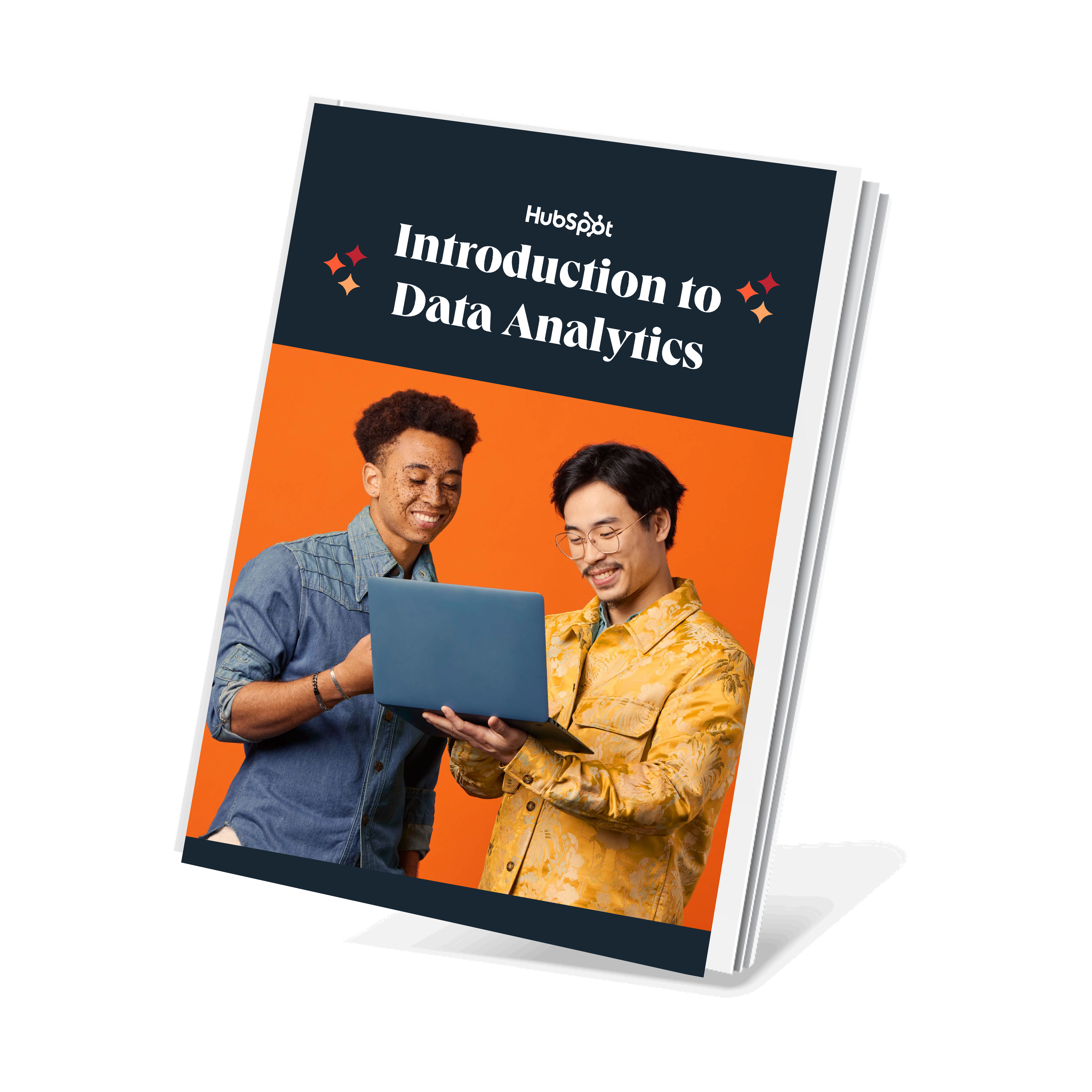
An Introduction to Data Analytics
Unlock the power of data and transform your business with HubSpot's comprehensive guide to data analytics.
- Fundamentals of data analytics
- Different types of data analytics
- Data visualization
- And more!
Download Free
All fields are required.
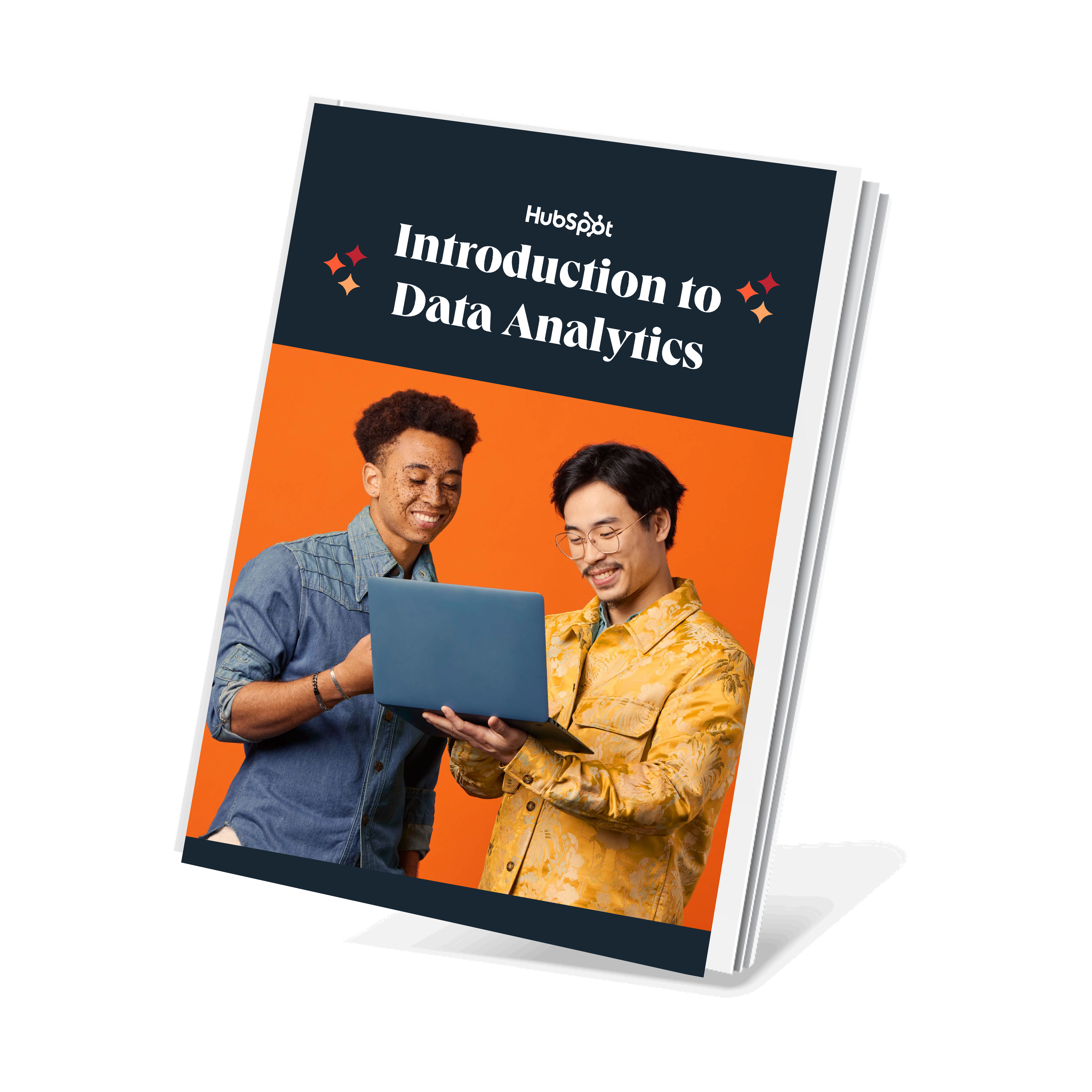
What Is AI Data Management?
To understand AI data management, we first need to understand data management as a broader concept. Data management can get complex, but it typically involves overseeing an organization's data throughout the data lifecycle. The different stages of the data lifecycle include:
- Collection.
- Access.
- Usage.
- Storage.
- Transfer.
- Deletion or destruction.
So, where does AI fit into the picture? AI data management means using artificial intelligence to achieve the above. Infusing AI into your data management can improve the quality, accessibility, and, when integrated correctly, security of your data.
On that latter point, whether AI-powered or not, you need to keep data security at the forefront of your mind throughout the process.
The Benefits of Using AI for Data Management
As I shared above, research shows that 100% of data experts are marching full speed ahead with their data management investments in 2024. It's rare that everyone agrees on something, especially regarding budget allocation — so I'm curious to know why.
Below, I explore some key benefits driving folks toward investing in AI data management solutions.
1. Wrangling Data From Multiple Sources
Nearly 40% of data leaders cite increasing volume and variety of data as the most significant technical challenge to completing their data strategy. In this “ever-evolving data landscape” (sorry, sorry, sorry!), it's hard to see the volume or variety slowing down anytime soon. As a result, many folks are turning to AI tech to wrangle and, therefore, make sense of all that company data.
Martin Fix, a co-lead of the AI Innovation Hub and technical director at Star, explains.
“AI can help to access and work with data from multiple and heterogeneous sources in a more simple way — as long as sufficient meta-data and technical interfaces are available which the AI can use,” says fix.
Fix also warns that without proper curation, AI will fail to provide real value.
2. Faster Decision Making
Alex Boquist, co-founder and CTO at Scout, a platform for building and deploying AI solutions, agrees with Fix regarding wrangling data from multiple sources.
“AI simplifies the challenge of integrating large datasets from multiple sources — like CRM systems, marketing platforms, and third-party APIs,” says Boquist. “For instance, AI-driven data pipelines can automate the aggregation and processing of sales and customer data in real time, providing dashboards with up-to-the-minute insights.”
The end benefit of streamlining your data integration and processing with AI? Faster decision-making. Beyond that, it reduces the delays between data collection and action. Boquist highlights that this is crucial for businesses that rely on dynamic insights for growth, such as ecommerce or SaaS companies.
3. Improving Efficiency and Reducing Cost
Having a single data management platform — or as minimal a tech stack as possible — reduces cost. AI data management tools can also save your team time by streamlining data-driven tasks. They can then reinvest this time into more creative, demanding, or higher-value tasks, improving efficiency.
An example of the latter would be streamlining data analysis through AI analytics. In this way, team members can process large amounts of data and uncover insights much faster than they could without this tech assist.
In the case of marketing, 44% of marketers agree AI is effective at performing data analysis — meanwhile, 70% use AI-powered reporting tools to improve their data analysis workflows.
4. Reducing Manual Task Time
Sticking with marketing, 75% of marketers use AI to reduce manual task time. The same HubSpot data finds that 86% say AI saves them 1+ hours daily by streamlining creative tasks. Part of that streamlining involves using AI tech to automate processes.
Beyond marketing, tasks like data classification and quality monitoring extend into most, if not all, industries.
Aside from this, “Automating compliance monitoring is another significant advantage,” according to Paul Massey, the chief revenue officer (CRO) at AI-driven recruitment app rTriibe.com. This helps “businesses maintain regulatory adherence without the manual burden.”
5. Security Threat Detection
Data security is integral to any data management process, but it matters more than ever in the age of AI. I recently penned an article about how AI impacts data security and privacy. Immuta's AI Security & Governance Report, which surveyed 700+ data experts from around the globe, was an invaluable resource.
From that research, I discovered that 80% of respondents agree AI makes data security more challenging. For context, 52% cite the possibility of AI attacks via threat actors as a significant risk. And 57% have witnessed an increase in AI-driven attacks in the last year.
So, what's the fix for this looming data security threat? After speaking to multiple experts for my article, it seems the best way to fight bad AI is with good AI.
Paul Massey agrees.
“AI's predictive threat detection capabilities are particularly valuable, allowing organizations to address potential security risks proactively before they become breaches,” says Massey. “Ultimately, AI enhances the efficiency, security, and quality of data management, enabling organizations to unlock the full potential of their data.”
AI Data Management Use Cases
Now that we know why folks are integrating AI into their data management systems and processes, I'd like to explore how they achieve this end. As with many areas of life, a one-size-fits-all solution often doesn't exist. But, these eight use cases should help you think about how — or even if — you should use AI data management in your organization.
1. Understand data lineage.
Data lineage is how you track the flow of data within your organization. When you understand data lineage, you can identify the data's origins, if it has changed over time, and where it fits in the data pipeline.
Doing this manually can be time and resource-intensive. So, it's unsurprising that, as Etai Mizrahi, CEO of data enablement platform Secoda, explains, folks are using AI to streamline this process.
“One of the most common use cases for Secoda AI is understanding data lineage,” says Mizrahi. “Users can ask the AI, ‘Can I drop this column?’ or ‘Can I drop this table without impacting downstream processes?’”
Mizrahi explains that AI platforms like Secoda index metadata, lineage, and tags to help identify potential downstream impacts. Secoda AI, in particular, can also visualize if a specific column is used in multiple tables and whether dropping it might disrupt the lineage graph.
Users can then search for that column, see the entire lineage, and notify the right owners directly. This proactive capability makes data management more efficient and transparent.
He adds, “It streamlines the entire process, helping teams avoid errors and maintain data integrity, all while speeding up decision-making. This level of real-time insight and control is what makes AI so valuable in day-to-day data management.”
2. Speed up data processing.
Traditionally, data processing was designated to the realm of the data scientist, where they'd collect, translate, and present data in a way other stakeholders could understand. Here's the thing: I don't think AI data processing can, or in fact should, replace data scientists. However, it can make them — and all data users — more effective at their role. Head of Marketing at Secoda Dexter Chu, shares an example below.
Chu explained that Secoda's AI data catalog helped Panasonic to efficiently process over 3TB of data weekly while ensuring governance through role-based access controls and automated lineage tracking. This improved data organization and built trust among stakeholders by proactively managing dependencies and sending automated notifications.
Pro tip: While AI tools can speed up data processing, I recommend keeping the right humans in the driver's seat. Any tool you use should empower top-tier talent, not replace them.
3. Streamline data cataloging.
Data cataloging involves building an organized inventory of all your data. As with many areas of data management, doing this manually is time-intensive. The good news? AI can save you time.
According to Dexter Chu, Secoda's AI-driven tools saved Hotel Oversight 80% of the time spent on manual data cataloging while improving data quality through proactive issue detection.
“The result is that businesses can scale their data operations with fewer errors, enhanced accuracy, and clearer governance, all while focusing more on decision-making and less on manual tasks,” he explains.
4. Monitor data pipelines in real time.
Your data pipeline is the path a group of data follows from one system to another. Aside from making it easier for you to track this data lineage over time, AI-driven data management platforms can also help you monitor it here and now.
CRO Paul Massey explains, “AI-powered data observability tools allow businesses to monitor their data pipelines in real time, catching anomalies or data drift, ensuring the continuous reliability of AI systems.”
According to Massey, that is just one use case where AI is proving indispensable for businesses looking to enhance their data management practices.
Pro tip: I might be biased, but HubSpot AI is one of the best CRMs for sales, marketing, and service teams. There are many ways to use the AI-powered platform to improve your data management process. My personal favorite is ChatSpot. You can ask ChatSpot questions and get quick data insights about your contacts from anywhere inside your CRM.
In my experience, this speeds up data analysis. It's also great for monitoring where your customers are in the sales pipeline. To top it off, you can enhance your data management capabilities further by integrating third-party data management apps into HubSpot. There are currently over 110 of these to choose from.
5. Classify and organize documents.
Michael Walker, chief marketing officer at AI agent platform SmythOS, states, “One of the key benefits of using AI in this field is the ability to automatically categorize, tag, and summarize vast amounts of data.”
Walker explains that this makes it much easier to navigate large repositories and quickly find relevant information. But what does this look like in practice?
“We have developed AI agents that automatically classify and organize documents, significantly reducing the time and effort required for manual data management,” says Walker. “These AI agents interpret and tag documents based on their content and context, enabling users to retrieve the information they need with greater speed and accuracy.”
6. Automate routine data tasks.
In the benefits section above, I covered that AI data management can reduce manual task time, improve efficiency, and help with faster decision-making. While many roads lead to these results, automating routine data tasks is perhaps the most effective.
Alex Boquist explains that “AI-powered workflows excel at automating repetitive tasks like data entry, validation, and transformation. A great example is automating financial reconciliations by matching transactions across bank feeds and accounting systems, flagging any discrepancies instantly.”
7. Enhance data quality and accuracy.
Informatica research finds that 41% of data leaders cite data quality and observability as top data management capabilities they want to invest in this year. Boquist explains how AI-driven solutions can help.
“AI ensures data quality by automating processes like deduplication, outlier detection, and data enrichment. For example, AI models can identify and correct inconsistencies in customer databases — such as outdated contact information — by cross-referencing multiple data sources automatically,” Boquist says.
The result? An improvement in the accuracy of analytics while ensuring data integrity. Further, AI can help to reduce the occurrence of manual errors. Given that bad inputs make for bad outputs, this is welcome news.
In this context, inaccurate data input can negatively impact data output and, thus, quality. The wrong data might also persuade companies to follow ill-fitting strategies. AI-enhanced tools can head these input issues off at the pass so your data stays clean, clear, and under control.
Pro tip: As someone who‘s been the only admin for an entire team of sales reps, I know how important data consistency is. Long story short? It’s almost impossible to even make sense of or use data effectively if every team member inputs data differently. That‘s why I love how HubSpot’s data quality software enforces consistency when reps input sales data.
8. Improve data governance and compliance.
If you‘re swimming in a sea of data, it makes sense to make it secure, accurate, and available to relevant stakeholders. Otherwise, you’re not thinking; you‘re sinking. That’s why data privacy and governance are top priorities for data leaders.
According to Martin Fix, “AI can help to get insights on data quality and consistency and to keep governance and regulations in mind (as it could be made to ‘know’ all of this and won't forget about it like humans do).”
Industry expert Paul Massey agrees: “By automating key processes such as data classification and quality monitoring, AI reduces the risk of human error and ensures compliance with regulations like GDPR and CCPA. It improves data lineage tracking, providing a clear view of how data moves across systems, which is vital for compliance.”
Beyond the Bells and Whistles
When it comes to AI integration in any area, there can be a lot of unnecessary bells and whistles. But in my experience, some of the more simple, dare I say, almost mundane, AI use cases are often the most impactful.
Regarding data management, I think tracking the flow of company data over time with AI can lead to efficiency. It also makes “data-driven decision making” (sorry, sorry, sorry, sorry!) accessible to even non-technical stakeholders. Another trickle-down impact of this seemingly simple use case is reducing data management-related support tickets, saving your technical team's time.
That said, the difference maker is whether your AI tool or solution of choice is simple enough for anyone to pick up and use — even if the backend has many layers of complexity.
I mention this because 39% of data leaders reported the increasing number of data consumers is the top technical obstacle to realizing their data strategy, according to Informatica. The crux? Many of these consumers need no-code data management solutions to perform their roles effectively.
And as Martin Fix warned earlier, without proper curation and sufficient meta-data and technical interfaces, AI will fail to provide real value.
I don‘t raise these points to rain on anyone’s AI parade. (If you want to know where I stand, personally, I'm skeptically optimistic about the tech.) I raise these points so you get genuine value from your AI data management investments. Now, good luck, and go get ‘em!
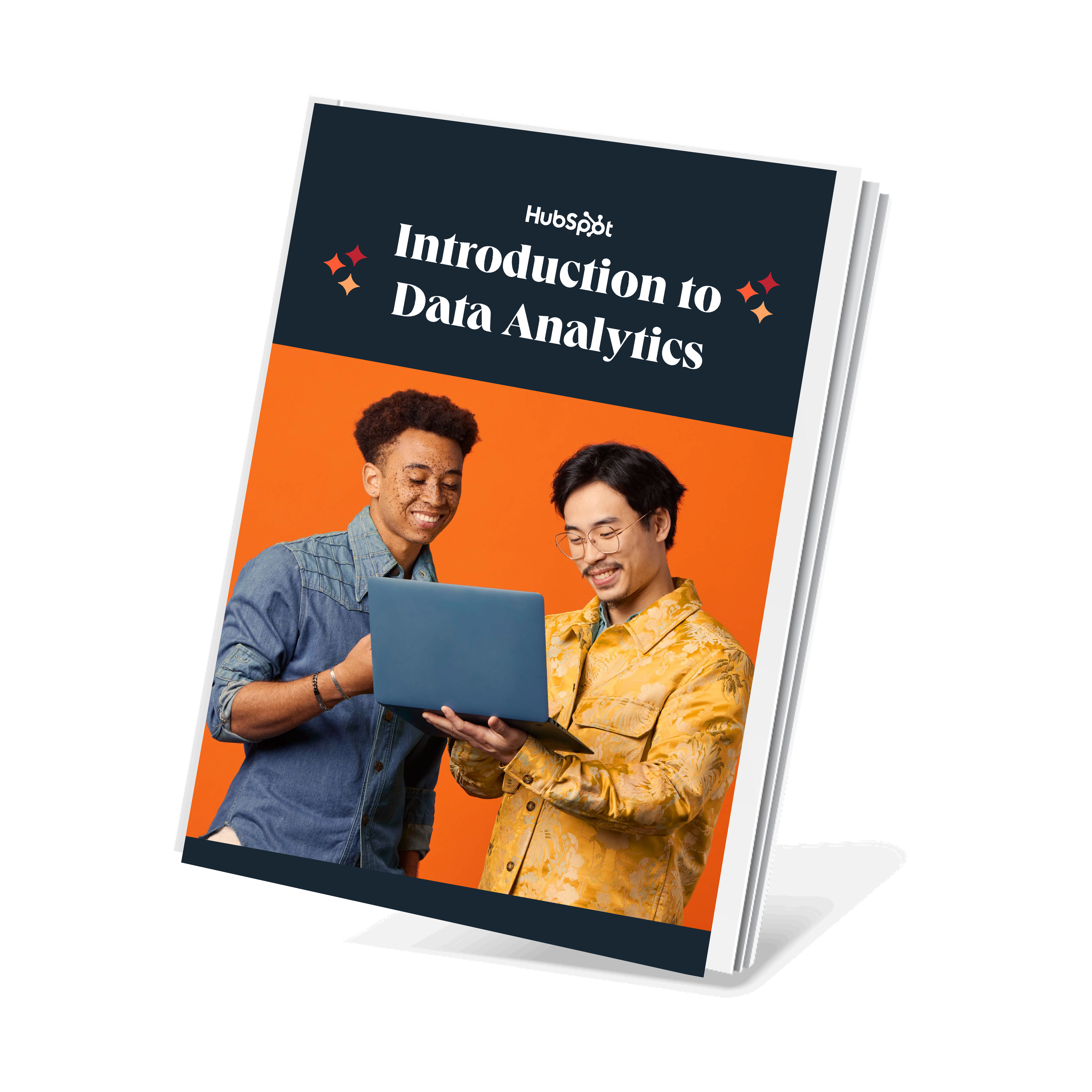
An Introduction to Data Analytics
Unlock the power of data and transform your business with HubSpot's comprehensive guide to data analytics.
- Fundamentals of data analytics
- Different types of data analytics
- Data visualization
- And more!
Download Free
All fields are required.
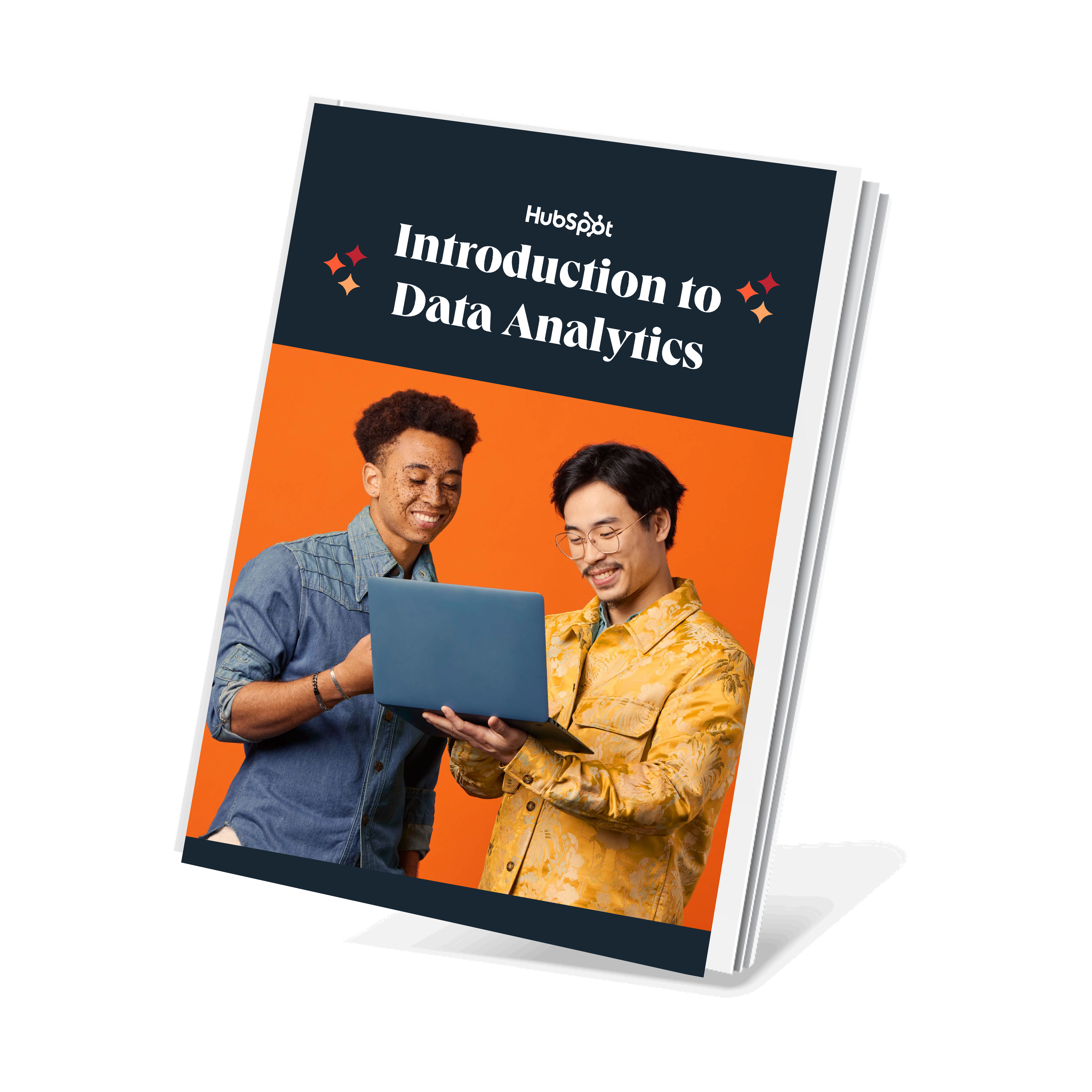