Ten years ago, most organizations saw data and analytics as a nice-to-have. Today, it is becoming a core business function across industries, with 97% of Fortune 1000 companies investing in data initiatives.
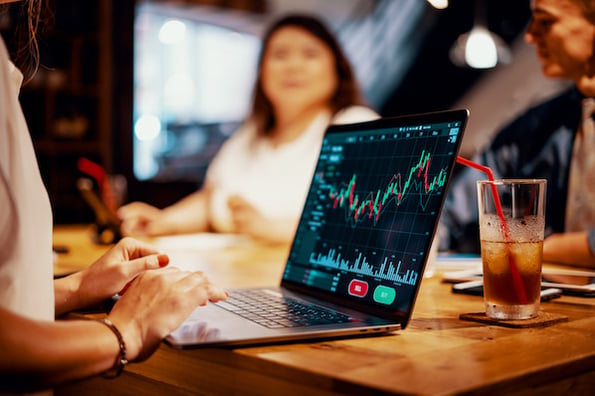
Data is a treasure trove of opportunity for those who know how to leverage its potential. But it’s also a black box of risk for those who don’t, both in terms of cybersecurity and compliance with ever-evolving regulations.
Knowing the following eleven trends will help companies stay shrewd and agile in the new era of data-driven business.
Data Trends
- The era of “big data” is fading out and the era of “small and wide data” is fading in.
- Data architectures are becoming composable.
- The realm of data analytics is becoming self-service.
- More departments are adopting data and analytics as a core business function.
- “Citizen Data Scientist” is an emerging role in companies.
- Data quality is becoming a major concern.
- Data standardization for AI workloads is on the rise.
- The demand for any-to-any integration is growing.
- How data is governed is becoming a key business concern.
- The issue of data security and compliance is gaining prominence.
- Customer data platforms will lose relevance.
1. The era of “big data” is fading out and the era of “small and wide data” is fading in.
In 2011, McKinsey & Company published an article stating that the new era of big data was upon us. What they meant was that companies tended to have a few, very complex systems that were starting to amass “mountains” of data.
Nowadays, due to the proliferation of software as a service (SaaS), many businesses are amassing several small “hills” of data across a wide landscape of applications. In 2015, the average number of SaaS applications being used by organizations worldwide was 8. In 2020, it was 80. And in 2021, it was 110.
The datasets from these cloud apps are smaller and simpler, yet more targeted and thus deliver better insights to decision-makers.
Gartner predicts that 70% of organizations will be paying the majority of their attention to small and wide data by 2025.
The SaaS boom is completely changing the way data is being handled within organizations, which leads us to the next trend.
2. Data architectures are becoming composable.
Among the cloud apps popping up everywhere are specialized tools for data integration, storage, and analysis. This fact has set in motion a trend towards the “composability” of data architectures, meaning that businesses pick and choose the tools that comprise their architectures at any given time, as dictated by their changing needs.
According to Gartner, “composable technology architecture is a foundation for digital enablement of business.” This trend was accelerated by COVID-19, which made organizational agility a key value for most organizations.
Just like big data is turning into small and wide data, vertical scaling of data stacks is shifting to horizontal scaling, whereby companies build “chains” or pathways for data processing instead of piling resources on top of existing systems. Here is an example of what a composable data architecture could look like:
It is also worth mentioning that composable data architectures are cheaper since they can be scaled at any pace and—because they are based on standardized applications provided by external vendors—they require less maintenance from in-house teams.
3. The realm of data analytics is becoming self-service.
Traditionally, dashboards have been built by IT teams that are decoupled from the business professionals who need to view them. When the business professionals require new information, they submit a request to their IT team, which can take anywhere from hours to months to fulfill.
This arrangement is a tall barrier to the flexibility that businesses increasingly need to maintain a competitive advantage.
Hence the rise of self-service analytics, whereby non-technical end users can set up data pipelines and customize dashboards independently of data engineers. Aside from pressure to shorten time to insights, this trend is being driven by three factors:
- Business intelligence (BI) tools are becoming more user-friendly.
- Non-technical end users have a desire to improve their data literacy.
- The appearance of no-code data integration tools.
The market for self-service BI tools is already extremely competitive, and is expected to grow 15% annually until 2026. There is also a clear push from vendors to make them as user-friendly as possible.
Below is an example of a user-friendly dashboard in Zoho Analytics:
A recent, global survey by Accenture of 9,000 employees from companies across industries indicates that many employees would like to be more data literate—37% stated that data literacy training would improve their efficiency and 22% felt that it would alleviate stress.
And finally, there are now several no-code data integration tools that let business users pull data from any source and send it to any destination for further processing and analysis.
4. More departments are adopting data and analytics as a core business function.
Data and analytics has the potential to guide improvement of just about any process in any business department. But until recently, its increase in importance has been most visible in marketing and sales departments.
Now, however, data and analytics is becoming a driving force behind the activities of other departments. This is reflected by the adoption of self-service BI tools across fields like HR, operations, finance, and even education.
Additionally, BI capabilities are now embedded in many department-specific tools, like recruitment platforms, which use AI to faster identify candidates, as well as demand planning software, which uses predictive analytics to help plan operations.
As data and analytics continues to become a core business function, companies will more frequently blend their data across departments to create an interconnected web of advanced insights.
5. “Citizen Data Scientist” is an emerging role in companies.
Data scientists and engineers are good at data, but they often don’t have the domain knowledge that is needed by individual departments to make that data actionable.
So, what we will see more and more of this year is “citizen data scientists,” i.e. professionals in non-data departments who have some knowledge of data analysis, but whose overall professional knowledge is better-aligned with their respective department. Here’s some tasks that a citizen data scientist would be able to perform:
These professionals know what data their departments need to track and how to visualize it using no-code tools. However, they themselves do not build the data models.
It is important to note the term “citizen data scientist,” coined by Gartner, will unlikely become the name of a new position advertised across job portals around the world. Instead, the responsibilities thereof will be written into the job descriptions of other positions.
Global companies like BP are already reaping great benefits from citizen data scientists.
6. Data quality is becoming a major concern.
As more users start working with data, the greater the potential is for mistakes in data to be proliferated to downstream systems.
Imagine a content manager whose Hubspot account is connected to Google Analytics (GA). A week after publishing a new blog article, they check their GA dashboard and, due to a common error in the measurement script, the article has double the views most articles have after their first week.
The manager then starts publishing more, similar articles, only to find that they are not producing the same results. Oops!
Situations like this have set in motion a twofold trend:
- Companies and employees are learning to pay more attention to data quality. Next time this content manager sees a dramatic spike in views in their GA dashboard, they will go back to their native Hubspot dashboard to make sure that the numbers match.
- Technologies for catching errors and anomalies are developing rapidly. Using AI, they mathematically define outliers by analyzing long time series. Anomaly detection tools now cater to a wide variety of business use cases, but since they work best when fed large datasets, they are not a silver bullet. The attention of users is therefore crucial.
7. Data standardization for AI workloads is on the rise.
The fact that we are getting our data from a greater number of simpler systems and tools means that it now comes in a wider variety of structures. This is a problem for AI-based analysis.
Calendar dates are a good example of why. One system may record them as MM.DD.YYYY while another may record them as DD.MM.YYYY. Humans can easily see past these variations, but machines can’t.
So, in order to make data analyzable by machines, it must be standardized or “transformed.” This is extremely important because nowadays there is a major boom in the use of AI-based applications across industries, most notably in IT and telecom, banking and finance, retail, healthcare, and marketing.
Traditionally, transformations have been the domain of developers, who execute them periodically on large volumes of data. This will remain relevant for many use cases, but due to the growing expanse of small and wide datasets that need to be processed quickly and frequently by non-technical professionals, no-code ETL tools such as Dataddo are the way forward.
8. The demand for any-to-any integration is growing.
As more and more specialized tools appear, so will the need to integrate them all with one another. This is because, ironically, the data integration tools that are now widely praised for eliminating data silos are actually new data silos.
To illustrate, let’s say that your accountant spends a lot of time working directly in Hubspot CRM, and that they want to extract invoicing data from Hubspot and pair it with external data for advanced insights. They can of course do this by sending the invoice data to a warehouse, blending it with the other data, and then viewing the results in a BI tool.
But this will eventually become a hassle, and the accountant will start wondering whether it’s possible to eliminate the BI tool as a silo and view all the data directly in Hubspot.
This is where any-to-any integration comes in. It empowers actors at all levels to create their own, single source of truth by sending data from any source or destination to any source or destination. Here’s an illustration of integrations among an ERP, CRM, website, ads campaigns, and social media platforms:
Any-to-any integration capability is so new that the terminology for it is not yet solidified, but it is sometimes referred to as data activation or reverse ETL.
There are very few tools for it on the market, but this will change within the next year or so.
9. How data is governed is becoming a key business concern.
The democratization of data, while empowering, has brought a wave of new challenges for enterprises.
According to Nick Halsey, CEO of data authorization company Okera, in an article in Forbes, these challenges include “too much data from too many sources [...] an unprecedented level of cyberattacks [... and] hybrid work environments that have employees moving between home and office, between personal and work devices.”
Companies are therefore facing mounting pressure to tackle the issue of data governance, which in practice means 2 things:
- Ensuring data quality.
- Ensuring data security.
If data is embedded in all decision-making processes, it needs to be complete and consistent. And if it’s being accessed by a growing number of users, they need to be the right users, at the right time and place.
This is forcing companies to craft data governance policies that seek a balance between centralization and decentralization. Centralization means better security and higher data quality, but less power from data; decentralization means greater potential for independent, informed decision-making, but higher risk of non-compliance and poor data quality.
Below is an illustration of the hub and spoke model of data governance:
The data governance market is expected to grow from 2.1 billion USD in 2020 to 5.7 billion USD by 2025—a nearly threefold increase!
10. The issue of data security and compliance is gaining prominence.
Whereas data governance is how companies choose to manage their data, data compliance is how they must manage their data. With data becoming more and more abundant, compliance with acts like GDPR in the EU and consumer data privacy laws in the US is a major issue.
The seriousness of this issue in Europe is reflected by the spiking annual GDPR fine totals. In 2020, fines totalled €306.3 million. In 2021, they exceeded €1 billion, with the largest fine to date—€746 million—being imposed on Amazon by the courts of Luxembourg.
There is no federal data privacy law in the US yet, and only three states have comprehensive consumer privacy laws in place, but most other states have some form of privacy legislation in the pipeline, so we can only expect that American enterprises will soon have no choice but to watch carefully what they collect and how they share it.
Gartner even predicts that by 2030, 50% of B2C businesses around the globe will stop retaining customer data due to impossible compliance costs.
11. Customer data platforms will lose relevance.
Customer data platforms (CDPs) are all-in-one software packages that collect and combine first- and third-party data from a variety of channels to give businesses a 360-degree view of their customers.
While they do enable integrations and visualizations of data, they are primarily designed for marketing use cases and come with preset data models that don’t offer the flexibility businesses will come to rely on.
This is a problem for two reasons:
- Businesses will need to be able to adapt their data architectures to meet quickly changing needs.
- Businesses will more and more want to integrate all their tools with one another to eliminate data silos.
CDPs will keep their place on markets where players have more fixed needs. But as cloud-based systems and specialized tools continue to proliferate, these all-in-one solutions are likely to stagnate on markets whose players need composability.
Seeing Clearly Through the Fog
The growing abundance of data is completely changing the way decisions are made across industries—by top management all the way down to frontline employees. And the tools out there today make it easier than ever and to build and maintain a data architecture that can stand up to market volatility.
Yes, improper data governance opens the door to regulatory non-compliance and cyber threats. But the benefits of data leverage far outweigh the difficulties that come with it.
The opportunities are massive, and businesses that don’t take them risk getting stuck in the fog.