pandas is a Python library that excels in data analysis and manipulation of relational data. Knowing how to determine the size, shape, and dimensions of a pandas DataFrame is crucial for extracting important information about its structure. These properties play a vital role in data analysis.
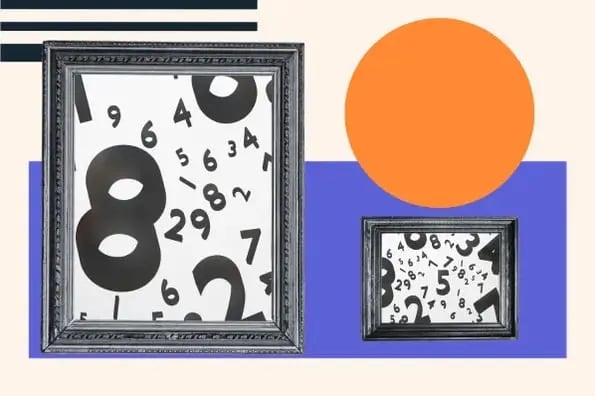
Selecting the most structured function for your analysis can make a significant difference in the resources required to complete a task. That all relies on the size of the DataFrame. For instance, if the DataFrame is small, a less efficient, adaptable function will be adequate. Meanwhile, a larger DataFrame requires a function that can handle complex data manipulation.
When you know these properties, you can make sound choices about which functions to use. Let's examine how to access these attributes.
Table of Contents
- What is a Pandas Data Frame?
- How to Find the Size of a Pandas DataFrame
- Is there a size limit for Pandas DataFrames?
- Syntax of Pandas DataFrame Size Attribute
- How to Find the Shape of a Pandas DataFrame
- How to Fetch the Dimensions of a Pandas DataFrame
What is a Pandas Data Frame?
A pandas DataFrame is a two-dimensional data structure similar to a table in a relational database or a spreadsheet. It consists of rows and columns. Each column can have a different data type (e.g., integer, float, string). However, all columns must have the same length.
Pandas is a popular data manipulation library in Python, and a DataFrame is one of its fundamental data structures. It provides various functions and methods to perform data analysis, data cleaning, and data transformation tasks efficiently.
How to Find the Size of a Pandas DataFrame
To determine the total number of cells in a pandas DataFrame, you can use the .size property. This property provides a useful insight into the amount of data contained within the DataFrame. It's calculated by multiplying the total number of rows by the total number of columns.
The following tutorials use a Major League Baseball (MLB) data set related to players' salaries. This information has been compiled by Kaggle. You can download the CSV file if you'd like to follow along with the examples.
To start, you import the pandas library and use the .read_csv() method to convert the data set into a DataFrame, which is assigned to the variable baseball_df:
import pandas as pd
baseball_df = pd.read_csv(‘./mlbSalaries.csv’)
You can confirm the DataFrame was created by using the .head() method, which provides a preview by pulling the first five rows of the DataFrame:
print(baseball_df.head())
The outcome of printing the .head() call is below.
You can see five rows of data on MLB players‘ salaries organized across five columns. In other words, you have a DataFrame. With large data sets, it’s more efficient to call a preview method, like .head, for quick confirmations. Trying to print the entire DataFrame takes much longer.
Now that you created the DataFrame, you can start to find its attributes. Let's start with the total number of cells:
print(baseball_df.size)
The output of the print statement is below.
You can see that your DataFrame has 11,700 cells. In other words, you have 11,700 values in your data set.
You can also save this value to a variable for future reference and calculations:
df_size = baseball_df.size
Given the current examination of size, you may wonder if there‘s a limit to how large a DataFrame can be. Let’s address this common question next.
Is there a size limit for Pandas DataFrames?
The short answer is yes. There is a size limit for pandas DataFrames, but it's so large you will likely never have to worry about it.
The long answer is the size limit for pandas DataFrames is 100 gigabytes (GB) of memory, instead of a set number of cells. In effect, this benchmark is so large that it would take an extraordinarily large data set to reach it.
To provide some context, you now know that the baseball_df DataFrame holds 11,700 values. To see what this translates to in memory, you can use the .info() method:
baseball_df.info(verbose = False)
The printout is below.
With 11,700 values, baseball_df hasn‘t even cracked 100 kilobytes yet. That’s less than one-tenth of a megabyte or one-thousandth of a GB. With a few conversions, it appears that you would need more than 12 billion data cells to approach the size limit with the current data set.
Syntax of Pandas DataFrame Size Attribute
Next, we’ll explore the DataFrame.size attribute, including how it’s written.
In this syntax, DataFrame refers to the name of the pandas DataFrame object of which you want to access the size attribute. The size attribute is used to return the total number of elements in the DataFrame. This is equal to the number of rows multiplied by the number of columns.
To access the size attribute, you would typically use it in conjunction with the DataFrame name, followed by a dot operator, and then the attribute name size.
For example, if you have a DataFrame named df, you can retrieve its size by using df.size. This will return a single integer value representing the total number of elements in the DataFrame.
Both missing and non-missing values are included in the DataFrame. The call df2=do.count ( ) will return pandas attribute values of N/A elements.
Now that you understand the size of your DataFrame, let's dive into how to find DataFrame structure.
How to Find the Shape of a Pandas DataFrame
Size alone doesn't reveal everything about your DataFrame. Another common attribute is the shape of a DataFrame.
The workflow for the .shape property is similar to the .size example:
print(baseball_df.shape)
The result of the print statement is below.
Here, the .shape property returns a tuple showing the DataFrame has 2,340 rows and 5 columns. A tuple is similar to a Python list in many respects; the biggest difference is that tuples are immutable, meaning they cannot be changed once declared.
Like lists, you can access each of the values at their corresponding index value:
baseball_df.shape[0]
# equals 2340
baseball_df.shape[1]
# equals 5
Using indexing, you can extract each of these values into variables to store and use in calculations. You can also use a Python shorthand known as unpacking to declare both variables on the same line:
row_count, column_count = baseball_df.shape
Let's examine the final attribute: dimensions.
How to Fetch the Dimensions of a Pandas DataFrame
Like the other two properties, accessing the dimensions of a pandas DataFrame is straightforward. Just use .ndim:
print(baseball_df.ndim)
The result of the print statement is below.
Here, you can see that the .ndim property returns an integer 2. This matches expectations because DataFrames are two-dimensional data structures, meaning they have rows and columns. If .ndim returned 1, then the baseball_df variable is a pandas Series, which is a one-dimensional data structure.
Pandas DataFrame size, shape, and dimensions power your strategic analysis.
The pandas library offers a range of tools for advanced data analysis. That includes the capability to remove insights from values and metadata for the entire data set.
By manipulating pandas, you can retrieve key attributes about a DataFrame, such as its size, shape, and dimensions. This can help speed up the metadata collection process and help you find the information you need effectively. These routes can provide a quick way to better understand your data set.