We‘ve all started at a data point in a spreadsheet and thought, "Why does this look so familiar?" There’s, of course, a reason: It's repeated data. Duplicate data takes up unnecessary storage space and slows down calculations. Removing duplicate data with Pandas can help you avoid this scenario in the future.
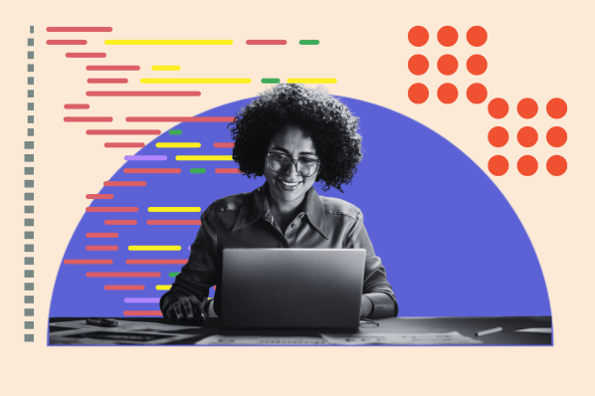
Pandas is a Python library designed to help developers manage structured data. Plus, Pandas supports data cleansing, which includes removing duplicated rows and columns.
This post will focus on the .duplicated and .drop_duplicates methods of the pandas library. Topics we'll cover include:
- How to Count the Number of Duplicated Rows in Pandas DataFrame
- Pandas .duplicated Arguments
- How to Drop Duplicate Rows in Pandas DataFrames
- How to Drop Duplicate Columns in Pandas DataFrames
How to Count the Number of Duplicated Rows in Pandas DataFrame
Let's create a simple example of duplicated rows in a Pandas DataFrame.
# create a example dataframe with duplicate rows
data = {
'Car': ['Forester', 'Outback', 'Outback', 'Outback', 'Forester'],
'Color': ['Brown', 'Silver', 'Silver', 'Silver', 'Black'],
'Interior': ['Black', 'Black', 'Black', 'Brown', 'Green']
The example allows us to see that the rows with index 1 and 2 have the same values for all three columns. Upon applying the .drop_duplicates( ) function, the first row is retained. The remaining duplicate rows are removed.
Before we start removing duplicate rows, it‘s wise to get a better idea of where the duplicates are in our data set. Then, we can decide how best to deal with them. Let’s get started.
[Video: Drop Duplicates from pandas DataFrame | How to Remove Repeated Row | All & Multiple Selected Columns ]
Below is a product inventory of kitchen cutlery and utensils. This will be our base DataFrame over the following tutorials:
With the .duplicated method, we can identify which rows are duplicates:
Here, we are calling .duplicated() on our DataFrame kitch_prod_df. Once executed, .duplicated() returns booleans organized into a pandas Series (also known as a column). We can inspect this output by printing to the terminal:
To interpret these results, think of .duplicated as asking, “Is this row duplicated?” The method looks through each row to determine if all the data present completely matches the values of any other row in the DataFrame. If it finds an exact match to another row, it returns True. In other words, it answers the question “Is this row duplicated?” with “Yes.”
This means that any rows that are marked False are unique. To return to the printout, this means we have four unique rows and two duplicate rows (“fork” and “spoon” at index positions 3 and 4).
The default behavior of .duplicated is to mark the second instance of a row as the duplicate (and any other instances that follow it). It considers the first row to contain the matching data as unique. However, you can modify this behavior by changing the arguments.
Let's review the arguments available for the .duplicated method and how you can adjust them to fit your needs.
Pandas .duplicated Arguments
1. The Keep Argument
Best for: Fine-tuning how .duplicated treats the origin row for any duplicates it finds.
Let's say we want to flag the origin row and any subsequent rows as duplicates. To do this, we turn to the keep argument.
kitch_prod_fd.duplicated(keep = False)
Here, we call .duplicated() with the argument keep set to the boolean False.
In effect, this tells .duplicated we don't want to “keep” any duplicates. Now, any two or more rows that share the same data will all be marked True in the output. We can see the original DataFrame and the boolean Series printouts below.
As expected, the first and second rows that were marked False in our last example are now marked True. Their data matches the duplicate rows in the fourth and fifth positions. This leaves us with two unique rows.
The default value for keep is “first.” This tells the .duplicated method that we want to mark the first and subsequent occurrences of duplicates as False and mark the last instance as True:
kitch_prod_df.duplicated(keep = 'first')
As “first” is the default keep argument, we don‘t need to include it when calling .duplicated(). However, it’s still important to understand how it works. We can confirm the method performed as expected by viewing the printout:
We can see the fourth and fifth rows are marked as duplicates. This matches the result when we executed .duplicated with no keep argument in the original example.
If you want to keep the last instance of a duplicate row, you can invert the default behavior of .duplicated by changing the argument to “last”:
Instead of marking the fourth and fifth rows, we have now identified the initial occurrences of the duplicate data as True.
In some scenarios, it makes sense to limit our search for duplicates to specific columns instead of the entire DataFrame. To achieve this, we use the subset argument.
2. The Subset Argument
Best for: Narrowing the scope of the rows that .duplicated inspects to suspect columns to produce more focused results.
Scanning the entire DataFrame isn‘t always practical, especially if it’s dozens of columns or hundreds of rows. If we identify columns where duplicates are likely to occur, we can pass the column names to .duplicated with the subset argument.
Here's the original DataFrame for reference:
In this code, we are checking the DataFrame for duplicates in the “department” column:
kitch_prod_df.duplicated(subset = 'department')
Here, we set the subset argument equal to “department” so that .duplicated() only examines the column matching that label. The output is below.
If we went off these results, we could conclude that our entire DataFrame is full of duplicate records. With context, we realize that all the products are under the “kitchen” department label. The subset argument allows us to expand our scope beyond a single column by using a Python list:
kitch_prod_fd.duplicated(subset = ['sku', 'department'])
This time, we are searching for duplicates in both the “sku” and “department” columns. Only if the data is the exact same in both columns will a row get marked as a duplicate. Even though every record shares the same department name, only the fourth and fifth rows are flagged as duplicates:
The keep and subset arguments are also available to us in the .drop_duplicates method, which we'll review in the following sections.
How to Drop Duplicate Rows in Pandas DataFrames
Best for: Removing rows you have determined are duplicates.
Now that we know where the duplicates are, we can use the .drop_duplicates method to remove them.
Let's explore the original DataFrame for reference:
By default, .drop_duplicates will remove the second and additional occurrences of any duplicate rows.
kitch_prod_fd.duplicated(inplace = True)
The results are below.
Here, .drop_duplicates has identified duplicates in the values of the “sku” column. The function dropped the rows belonging to those repeat values — the fourth and fifth rows.
This video from DataDaft provides a live demonstration of the .drop_duplicates method.
[Video: How to Remove Duplicate Rows From a Data Frame in Pandas (Python) ]
Now, let's look at how we can modify this approach to remove duplicate columns.
How to Drop Duplicate Columns in Pandas DataFrames
Best for: Removing columns you have determined are duplicates of other columns with only a slight adjustment to the syntax.
You may encounter columns that hold identical values that need to be removed. However, .drop_duplicates only works for rows.
Fortunately, Python offers a trick for this use case. In short, we will temporarily make our columns into rows.
Here's our DataFrame, now including a second column labeled “products” that contains the same values as the original “product” column.
The .T property allows us to invert the axis of our DataFrame. You can see the output of printing kitch_prod_df.T to the terminal below.
Now, .drop_duplicates can look through our DataFrame and remove duplicate rows, because the columns are the rows.
no_dup_columns = kitch_prod_df.T.drop_duplicates().T
Here, we are inverting the kitch_prod_df DataFrame with the .T property. We can call .drop_duplicates() to examine the rows. By default, .drop_duplicates() will keep the first instance of a duplicate row and remove any others.
We are then removing the inversion with the second .T property. The DataFrame will then display normally and save it to a new variable no_dup_columns.
The output of printing the new DataFrame is below.
Here, we can see the duplicate column “products” is now removed from our DataFrame. Even though the column names between “product” and “products” were different, their values were identical.
We can modify how .drop_duplicates removes duplicate columns through the keep and subset arguments.
Getting Started
In the world of data analysis, good decision-making relies heavily on good data. However, not all data is created equal. It's essential to ensure that your data is accurate and reliable. Data cleansing is important to this process, which involves removing duplicate information.
By manipulating the .duplicated( ) and .drop_duplicates( ) methods within Pandas, you can effortlessly locate and remove any duplicated data. You can then be confident that you're analyzing clean, reliable data. Soon, you can make better-informed decisions to improve business outcomes.